Welcome to this introduction to TensorRT, our platform for deep learning inference. You will learn how to deploy a deep learning application onto a GPU, increasing throughput and reducing latency during inference. TensorRT provides APIs and parsers to import trained models from all major deep learning frameworks. It then generates optimized runtime engines deployable in the datacenter as well as in automotive and embedded environments. Applications deployed on GPUs with TensorRT perform up to 40x faster than CPU-only platforms.
This tutorial uses a C++ example to walk you through importing an ONNX model into TensorRT, applying optimizations, and generating a high-performance runtime engine for the datacenter environment. TensorRT supports both C++ and Python and developers using either will find this workflow discussion useful. If you prefer to use Python, refer to the API here in the TensorRT documentation.
Deep learning applies to a wide range of applications such as natural language processing, recommender systems, image, and video analysis.  As more applications use deep learning in production, demands on accuracy and performance has led to strong growth in model complexity and size. Safety-critical applications such as automotive place strict requirements on throughput and latency expected from deep learning models. The same holds true for some consumer applications, including recommenders. TensorRT is designed to help deploy deep learning for these use cases. With support for every major framework, TensorRT helps process large amounts of data with low latency through powerful optimizations, use of reduced precision, and efficient memory use.
Following along requires a computer with a CUDA-capable GPU or a cloud instance with GPUs and an installation of TensorRT. On Linux, the easiest place to get started is by downloading the TensorRT container from the Nvidia Container Registry, part of the Nvidia GPU Cloud.
A Simple TensorRT Example
This example has three steps: importing a pre-trained image classification model into TensorRT, applying optimizations and generating an engine, and performing inference on the GPU, as figure 1 shows.

The first step is to import the model, which includes loading it from a saved file on disk and converting it to a TensorRT network from its native framework or format. Our example loads the model in ONNX format from the ONNX model zoo. ONNX is a standard for representing deep learning models enabling them to be transferred between frameworks. (Many frameworks such as Caffe2, Chainer, CNTK, PaddlePaddle, PyTorch, and MXNet support the ONNX format). Next, an optimized TensorRT engine is built based on the input model, target GPU platform, and other configuration parameters specified. The last step is to provide input data to the TensorRT engine to perform inference. The sample uses input data bundled with the model from the ONNX model zoo to perform inference.
The sample uses the following components in TensorRTÂ to perform above steps:
- ONNX parser: takes a trained model in ONNX format as input and populates a network object in TensorRT
- Builder: takes a network in TensorRT and generates an engine that is optimized for the target platform
- Engine: takes input data, performs inferences and emits inference output
- Logger: object associated with the builder and engine to capture errors, warnings and other information during the build and inference phases
Let’s apply this to import a pretrained ResNet50 model in ONNX format and perform inference in TensorRT. Start with the TensorRT container from NGC registry to get all components pre-installed and ready to go.  Or install using step-by-step installation instructions in the TensorRT Installation Guide. Once you have installed TensorRT successfully, run the commands below to download everything needed to run this sample (the example code, test input data, reference outputs), update dependencies, and compile the application with the makefile provided.
>> git clone https://github.com/parallel-forall/code-samples.git >> cd code-samples/posts/TensorRT-introduction >> wget https://s3.amazonaws.com/onnx-model-zoo/resnet/resnet50v2/resnet50v2.tar.gz // Get ONNX model and test data >> tar xvf resnet50v2.tar.gz # unpack model data into resnet50v2 folder >> apt-get update >> apt install libprotobuf-dev protobuf-compiler     # install protobuf to read the input data which is in .pb format >> git clone --recursive https://github.com/onnx/onnx.git # pull onnx repository from github >> cd onnx >> cmake . # compile and install onnx >> make install -j12 >> cd .. >> make # compile the TensorRT C++ sample code
Let’s begin with a simplified version of the application, simpleONNX_1.cpp, and build on it. Subsequent versions are called simpleONNX_2.cpp and simpleONNX.cpp available in the same folder.
Run the sample with the trained model and input data passed as inputs. The data is provided as an ONNX protobuf file. The sample compares output generated from TensorRT with reference values available as onnx pb files in the same folder, and summarizes the result on the prompt. It can take a few seconds to import the ResNet50v2 ONNX model and generate the engine. We are using TensorRT 5 on a Turing T4 GPU, performance on your might vary based on your setup.
>> ./simpleOnnx_1 resnet50v2/resnet50v2.onnx resnet50v2/test_data_set_0/input_0.pb # sample expects output reference values in resnet50v2/test_data_set_0/output_0.pb ... INFO: Formats and tactics selection completed in 27.3666 seconds. INFO: After reformat layers: 75 layers INFO: Block size 3211264 INFO: Block size 3211264 INFO: Block size 3211264 INFO: Block size 1605632 INFO: Block size 0 INFO: Total Activation Memory: 11239424 INFO: Data initialization and engine generation completed in 0.148307 seconds. OK
And that’s it, you have a simple application that is optimized with TensorRT and running on your GPU!
Brief Code Walkthrough
Let’s review few key code snippets used in the sample above, the complete code is also included at the end of this section for reference.
The main function in the following code sample starts by declaring a CUDA engine to hold the network definition and trained parameters. The engine is generated in the createCudaEngine
 function that takes the path to the ONNX model as input.
// Declare CUDA engine unique_ptr<ICudaEngine, Destroy<ICudaEngine>> engine{nullptr}; ... // Create CUDA Engine engine.reset(createCudaEngine(onnxModelPath));
The createCudaEngine
 function parses the ONNX model and holds it in the network object. The builder converts the network into a TensorRT engine. The code snippet below demonstrates that.
ICudaEngine* createCudaEngine(string const& onnxModelPath) { unique_ptr<IBuilder, Destroy<IBuilder>> builder{createInferBuilder(gLogger)}; unique_ptr<INetworkDefinition, Destroy<INetworkDefinition>> network{builder->createNetwork()}; unique_ptr<nvonnxparser::IParser, Destroy<nvonnxparser::IParser>> parser{nvonnxparser::createParser(*network, gLogger)}; if (!parser->parseFromFile(onnxModelPath.c_str(), static_cast<int>(ILogger::Severity::kINFO))) { cout << "ERROR: could not parse input engine." << endl; return nullptr; } return builder->buildCudaEngine(*network); // build and return TensorRT engine }
Once an engine has been created, create an execution context to hold intermediate activation values generated during inference. The code below shows how to create the execution context.
// declaring execution context unique_ptr<IExecutionContext, Destroy<IExecutionContext>> context{nullptr}; ... // create execution context context.reset(engine->createExecutionContext());
This application places inference requests on the GPU asynchronously in the function launchInference
 shown below. Inputs are copied from host (CPU) to device (GPU) within launchInference
, inference then performed with the enqueue
 function, and results copied back asynchronously. The sample uses CUDA streams to manage asynchronous work on the GPU. Asynchronous inference execution generally increases performance by overlapping compute as it maximizes GPU utilization. The enqueue
 function places inference requests on CUDA streams and takes runtime batch size, pointers to input, output, plus the CUDA stream to be used for kernel execution as input.  Asynchronous data transfers are performed from the host to device and vice versa using cudaMemcpyAsync
.
void launchInference(IExecutionContext* context, cudaStream_t stream, vector<float> const& inputTensor, vector<float>& outputTensor, void** bindings, int batchSize) { int inputId = getBindingInputIndex(context); cudaMemcpyAsync(bindings[inputId], inputTensor.data(), inputTensor.size() * sizeof(float), cudaMemcpyHostToDevice, stream); context->enqueue(batchSize, bindings, stream, nullptr); cudaMemcpyAsync(outputTensor.data(), bindings[1 - inputId], outputTensor.size() * sizeof(float), cudaMemcpyDeviceToHost, stream); }
Using the cudaStreamSynchronize
 function after calling launchInference
 ensures GPU computations complete before the results are accessed The number of inputs and outputs, as well as the value and dimension of each, can be queried using functions from the ICudaEngine class. The sample finally compares reference output with TensorRT-generated inferences and prints discrepancies to the prompt.
Find more information about classes used in the TensorRT class reference manual. The complete code snippet is below.
#include "cudaWrapper.h" #include "ioHelper.h" #include <NvInfer.h> #include <NvOnnxParser.h> #include <algorithm> #include <cassert> #include <iostream> #include <memory> #include <string> #include <vector> using namespace nvinfer1; using namespace std; using namespace cudawrapper; static Logger gLogger; // Maxmimum absolute tolerance for output tensor comparison against reference constexpr double ABS_EPSILON = 0.005; // Maxmimum relative tolerance for output tensor comparison against reference constexpr double REL_EPSILON = 0.05; ICudaEngine* createCudaEngine(string const& onnxModelPath, int batchSize) { unique_ptr<IBuilder, Destroy> builder{createInferBuilder(gLogger)}; unique_ptr<INetworkDefinition, Destroy> network{builder->createNetwork()}; unique_ptr<nvonnxparser::IParser, Destroy> parser{nvonnxparser::createParser(*network, gLogger)}; if (!parser->parseFromFile(onnxModelPath.c_str(), static_cast(ILogger::Severity::kINFO))) { cout << "ERROR: could not parse input engine." << endl; return nullptr; } return builder->buildCudaEngine(*network); // build and return TensorRT engine static int getBindingInputIndex(IExecutionContext* context) { return !context->getEngine().bindingIsInput(0); // 0 (false) if bindingIsInput(0), 1 (true) otherwise } void launchInference(IExecutionContext* context, cudaStream_t stream, vector const& inputTensor, vector& outputTensor, void** bindings, int batchSize) { int inputId = getBindingInputIndex(context); cudaMemcpyAsync(bindings[inputId], inputTensor.data(), inputTensor.size() * sizeof(float), cudaMemcpyHostToDevice, stream); context->enqueue(batchSize, bindings, stream, nullptr); cudaMemcpyAsync(outputTensor.data(), bindings[1 - inputId], outputTensor.size() * sizeof(float), cudaMemcpyDeviceToHost, stream); } void softmax(vector& tensor, int batchSize) { size_t batchElements = tensor.size() / batchSize; for (int i = 0; i < batchSize; ++i) { float* batchVector = &tensor[i * batchElements]; double maxValue = *max_element(batchVector, batchVector + batchElements); double expSum = accumulate(batchVector, batchVector + batchElements, 0.0, [=](double acc, float value) { return acc + exp(value - maxValue); }); transform(batchVector, batchVector + batchElements, batchVector, [=](float input) { return static_cast(std::exp(input - maxValue) / expSum); }); } } void verifyOutput(vector const& outputTensor, vector const& referenceTensor) { for (size_t i = 0; i < referenceTensor.size(); ++i) { double reference = static_cast(referenceTensor[i]); // Check absolute and relative tolerance if (abs(outputTensor[i] - reference) > max(abs(reference) * REL_EPSILON, ABS_EPSILON)) { cout << "ERROR: mismatch at position " << i; cout << " expected " << reference << ", but was " << outputTensor[i] << endl; return; } } cout << "OK" << endl; } int main(int argc, char* argv[]) { // declaring cuda engine unique_ptr<ICudaEngine, Destroy> engine{nullptr}; // declaring execution context unique_ptr<IExecutionContext, Destroy> context{nullptr}; vector inputTensor; vector outputTensor; vector referenceTensor; void* bindings[2]{0}; vector inputFiles; CudaStream stream; if (argc != 3) { cout << "usage: " << argv[0] << " " << endl; return 1; } string onnxModelPath(argv[1]); inputFiles.push_back(string{argv[2]}); int batchSize = inputFiles.size(); // Create Cuda Engine engine.reset(createCudaEngine(onnxModelPath, batchSize)); if (!engine) return 1; // Assume networks takes exactly 1 input tensor and outputs 1 tensor assert(engine->getNbBindings() == 2); assert(engine->bindingIsInput(0) ^ engine->bindingIsInput(1)); for (int i = 0; i < engine->getNbBindings(); ++i) { Dims dims{engine->getBindingDimensions(i)}; size_t size = accumulate(dims.d, dims.d + dims.nbDims, batchSize, multiplies()); // Create CUDA buffer for Tensor cudaMalloc(&bindings[i], size * sizeof(float)); // Resize CPU buffers to fit Tensor if (engine->bindingIsInput(i)) inputTensor.resize(size); else outputTensor.resize(size); } // Read input tensor from ONNX file if (readTensor(inputFiles, inputTensor) != inputTensor.size()) { cout << "Couldn't read input Tensor" << endl; return 1; } // Create Execution Context context.reset(engine->createExecutionContext()); launchInference(context.get(), stream, inputTensor, outputTensor, bindings, batchSize); // wait until the work is finished cudaStreamSynchronize(stream); vector referenceFiles; for (string path : inputFiles) referenceFiles.push_back(path.replace(path.rfind("input"), 5, "output")); // try to read and compare against reference tensor from protobuf file referenceTensor.resize(outputTensor.size()); if (readTensor(referenceFiles, referenceTensor) != referenceTensor.size()) { cout << "Couldn't read reference Tensor" << endl; return 1; } // Apply a softmax on the CPU to create a normalized distribution suitable for measuring relative error in probabilities. softmax(outputTensor, batchSize); softmax(referenceTensor, batchSize); verifyOutput(outputTensor, referenceTensor); for (void* ptr : bindings) cudaFree(ptr); return 0; }
Batch your Inputs
This application example expects a single input and returns output after performing inference on it. Real applications commonly batch inputs to achieve higher performance and efficiency. A batch of inputs identical in shape and size can be computed on different layers of the neural network in parallel. Larger batches generally enable more efficient use of GPU resources. For example, batch sizes using multiples of 32 may be particularly fast and efficient on V100 and Tesla T4 GPUs because TensorRT can use special kernels for matrix multiply and fully connected layers that leverage Tensor Cores.
Pass the images to the application on the command line using the code below. The number of images (.pb files) passed as input arguments on the command line determine the batch size in this sample. Use test_data_set_*
to take all the input_0.pb files from all the directories.  Instead of reading just one input, the command below reads all inputs available in the folders. Currently, the downloaded data has three input directories, so batch size will be 3. This version of the sample profiles the application and prints the result to the prompt—more on that in the next section.
>> ./simpleOnnx_2 resnet50v2/resnet50v2.onnx resnet50v2/test_data_set_*/input_0.pb # use all available test data sets ... INFO: After reformat layers: 75 layers INFO: Block size 9633792 INFO: Block size 9633792 INFO: Block size 9633792 INFO: Block size 4816896 INFO: Block size 0 INFO: Total Activation Memory: 33718272 INFO: Data initialization and engine generation completed in 0.120244 seconds. Inference batch size 3 average over 10 runs is 14.5005ms OK
Since we want to process multiple images in one inference pass, we made a couple of changes to our application. First, collect all images (.pb files) in a loop to use as input in the application:
129c158,160 < input_files.push_back(string{argv[2]}); --- > for (int i = 2; i < argc; ++i) > input_files.push_back(string{argv[i]});
Next, specify the maximum batch size that a TensorRT engine expects using the setMaxBatchSize
 function. The builder then generates an engine tuned for that batch size by choosing algorithms that maximize its performance on the target platform. While the engine will not accept larger batch sizes, using smaller batch sizes at runtime is allowed. The choice of maxBatchSize
 value depends on the application as well as the expected inference traffic (e.g number of images) at any given time.  A common practice is to build multiple engines optimized for different batch sizes (using different maxBatchSize values) then choose the most optimized engine at runtime. When not specified, the default batch size is one, meaning that the engine will not process batch sizes greater than one. Set this parameter as shown in the code snippet below.
// Build TensorRT engine optimized based on batch size of input data provided builder->setMaxBatchSize(batchSize);
Profile it!
Now that you’ve seen a simple example, let’s discuss how to measure its performance. The simplest performance measurement for network inference is the time elapsed between an input being presented to the network and an output being returned, referred to as latency.  For many embedded applications, latency is a safety-critical requirement while consumer applications require quality-of-service. Lower latencies make these applications better. Our sample measures the average latency of an application using time stamps on the GPU. There are many ways to profile you application in CUDA, and this post provides a good introduction to the commonly used methods and their pros-cons.
CUDA offers a lightweight event API to create and destroy events, record events as well as calculate the time between them. The application can record events in the CUDA stream, one before initiating inference and another after the inference completes, shown in the code below. In some cases you might care about including the time it takes to transfer data between the GPU and CPU before inference initiates and after inference completes. Techniques exist to pre-fetch data to the GPU as well as overlap compute with data transfers that can significantly hide data transfer overhead. The function cudaEventElapsedTime
 measures the time between these 2 events being encountered in the CUDA stream.
Use the code at the beginning of the last section to run this sample and review profiling output.  To profile the application, we wrap the inference launch within the function doInference in simpleONNX_2.cpp. Note the updated function call below:
165,167c196
< Â Â Â Â Â Â Â Â launchInference(context.get(), stream, inputTensor, outputTensor, bindings, batchSize);
< Â Â Â Â Â Â Â Â // wait until the work is finished
< Â Â Â Â Â Â Â Â cudaStreamSynchronize(stream);
---
> Â Â Â Â Â Â Â Â doInference(context.get(), stream, inputTensor, outputTensor, bindings, batchSize);
Calculate latency within doInference
 as follows:
// Number of times we run inference to calculate average time constexpr int ITERATIONS = 10; ... void doInference(IExecutionContext* context, cudaStream_t stream, vector const& inputTensor, vector& outputTensor, void** bindings, int batchSize) { CudaEvent start; CudaEvent end; double totalTime = 0.0; for (int i = 0; i < ITERATIONS; ++i) { float elapsedTime; // Measure time it takes to copy input to GPU, run inference and move output back to CPU cudaEventRecord(start, stream); launchInference(context, stream, inputTensor, outputTensor, bindings, batchSize); cudaEventRecord(end, stream); // wait until the work is finished cudaStreamSynchronize(stream); cudaEventElapsedTime(&elapsedTime, start, end); totalTime += elapsedTime; } cout << "Inference batch size " << batchSize << " average over " << ITERATIONS << " runs is " << totalTime / ITERATIONS << "ms" << endl; }
Many applications perform inferences on large amounts of input data offline. The maximum number of inferences possible per second, known as throughput, is a valuable metric for these applications. You measure throughput by generating optimized engines for larger specific batch sizes, run inference, and measure the number of batches that can be processed per second. Use the number of batches per second and batch size to calculate the the number of inferences per second. We will leave that as a further exercise for you to try.
Optimize your Application
Now that you know how to run inference in batches and profile your application, let’s optimize it. TensorRT’s key strength is its flexibility and use of techniques including mixed precision, efficient optimizations on all GPU platforms, and the ability to optimize across a wide range of models types. In this section, we describe a few techniques to increase throughput and reduce latency from applications. The TensorRT best practices guide provides a comprehensive description of the techniques available and how to take advantage of them. Let’s look at a few common techniques below.
Using Mixed Precision Computation
TensorRT uses FP32 algorithms for performing inference to obtain the highest possible inference accuracy. However, you can use FP16 and INT8 precisions for inference with minimal impact to accuracy of results in many cases. Using reduced precision to represent models enables you to fit larger models in memory and achieve higher performance given lower data transfer requirements for reduced precision. You can also mix computations in FP32 and FP16 precision with TensorRT, referred to as mixed precision, or use INT8 quantized precision for weights, activations and execute layers.
Enabling FP16 kernels is as simple as setting the setFp16Mode
 parameter to true for devices that support fast FP16 math.
builder->setFp16Mode(builder->platformHasFastFp16());
The setFp16Mode
 parameter indicates to the builder that a lower precision is acceptable for computations and TensorRT will use FP16 optimized kernels if they perform better with the chosen configuration and target platform. With this mode turned on, weights can be specified in FP16 or FP32, and they will be converted automatically to the appropriate precision for the computation. You also have the flexibility of specifying 16-bit floating point data type for input and output tensors. We will leave this as an exercise for the user.
Set the Maximum Workspace Size
TensorRT allows user to increase GPU memory footprint during the engine building phase with the setMaxWorkspaceSize
 parameter. Increasing the limit may affect the number of applications that could share the GPU at the same time. Setting this limit too low may filter out several algorithms and thus create a sub-optimal engine. TensorRT allocates just the memory required even if the amount set in IBuilder::setMaxWorkspaceSize()
 is much higher. Applications should therefore allow the TensorRT builder as much workspace as they can afford. TensorRT allocates no more than this and typically less.
In our sample we use 1GB, that lets TensorRT pick any algorithm available.
// Allow TensorRT to use up to 1GB of GPU memory for tactic selection constexpr size_t MAX_WORKSPACE_SIZE = 1ULL << 30; // 1 GB worked well for this sample ... // set builder flag builder->setMaxWorkspaceSize(MAX_WORKSPACE_SIZE);
Reuse the TensorRT Engine
When building the engine, the builder object selects the most optimized kernels for the chosen platform and configuration. Building the engine from a network definition file can be time consuming and should not be repeated each time we need to perform inference unless the model, platform, or configuration changes. You can transform the format of the engine after generation and store on disk for reuse later, known as serializing the engine. Deserializing occurs when you load the engine from disk into memory and continue to use it for inference. The steps are outlined in figure 2.
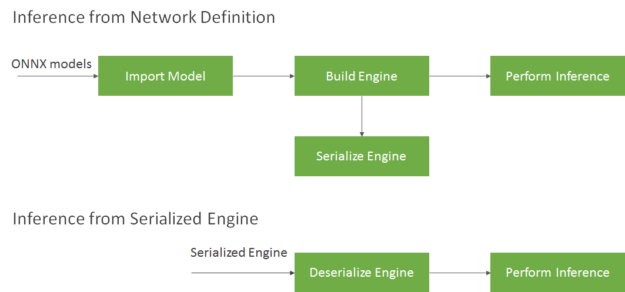
The runtime
 object deserializes the engine.
Instead of creating the engine each time, simpleOnnx.cpp contains the getCudaEngine
 function to load and use an engine if it exists. If the engine is not available, it creates and saves the engine in the current directory with the name onnx_filename.engine
. Our sample picks this engine if it is available in the current directory before trying to build a new engine. To force that a new engine is built with updated configuration and parameters, use the make clean_engines
 command to delete all existing serialized engines stored on disk before re-running the sample.
164c198
< Â Â Â Â Â Â Â Â engine.reset(createCudaEngine(onnxModelPath));
---
> Â Â Â Â Â Â Â Â engine.reset(getCudaEngine(onnxModelPath));
See the getCudaEngine
function below.
ICudaEngine* getCudaEngine(string const& onnxModelPath, int batchSize) { string enginePath{getBasename(onnxModelPath) + "_batch" + to_string(batchSize) + ".engine"}; ICudaEngine* engine{nullptr}; string buffer = readBuffer(enginePath); if (buffer.size()) { // try to deserialize engine unique_ptr<IRuntime, Destroy> runtime{createInferRuntime(gLogger)}; engine = runtime->deserializeCudaEngine(buffer.data(), buffer.size(), nullptr); } if (!engine) { // Fallback to creating engine from scratch engine = createCudaEngine(onnxModelPath, batchSize); if (engine) { unique_ptr<IHostMemory, Destroy> engine_plan{engine->serialize()}; // try to save engine for future uses writeBuffer(engine_plan->data(), engine_plan->size(), enginePath); } } return engine; }
Let’s use this saved engine with different batch sizes. The code below will take input data, repeat it as many times as your batch size variable, and then pass this appended input to our sample.
>> for x in `seq 1 $BATCH_SIZE`; do echo resnet50v2/test_data_set_0/input_0.pb ; done | xargs ./simpleOnnx resnet50v2/resnet50v2.onnx ... INFO: Glob Size is 51388144 bytes. INFO: Added linear block of size 102760448 INFO: Added linear block of size 102760448 INFO: Added linear block of size 102760448 INFO: Added linear block of size 102760448 INFO: Deserialize required 1270729 microseconds. Inference batch size 4 average over 10 runs is 3.99671112512ms OK
That’s it! You’ve now learned the basics of how to optimize a deep learning application for inference using TensorRT. Many other optimization techniques exist, such as overlapping data transfer between CPU – GPU with compute, and using INT8 precision, that can help you achieve even higher inference performance. By moving inference of this introductory sample from the CPU to GPU with TensorRT, we measured over 100x1 lower latency.
Exercises
Hopefully this sample has familiarized you with the key concepts needed to get amazing performance with TensorRT. Below are some exercises for you to apply what you have learned, use other models, and explore the impact of design and performance tradeoffs by changing parameters we have introduced in the blog.
- If you have not installed TensorRT, refer to the TensorRT Installation Guide for installation requirements, a list of what is included in the TensorRT package, and step-by-step instructions for installing TensorRT. The TensorRT support matrix provides a look into supported features and software for TensorRT APIs, parsers, and layers. While this example used C++, TensorRT provides both C++ and Python APIs. Refer to the APIs and well as see Python and C++ code examples in the TensorRT Developers Guide to run the sample included in this article.
- Try out different ONNX models, such as Squeezenet or Alexnet. These models in ONNX format and test data can be found here GitHub: ONNX Models.
- Change the allowable precision with the parameter
setFp16Mode
 to true/false for above models and profile the applications to see difference in performance - Change the batch size used at run time for inference and see how that impacts performance (latency, throughput) of your model and dataset
Learn More
You can find numerous resources to help you accelerate applications for image/video, speech and  recommender applications. These range from code samples, self-paced Deep Learning Institute labs and tutorials to developers tools for profiling and debugging applications. Below are are handy resources for you to learn more.
One topic that not covered in this tutorial is performing inference accurately in TensorRT with INT8 precision. TensorRT automatically converts an FP32 network for deployment with INT8 reduced precision while minimizing accuracy loss. In order to achieve this goal, TensorRT uses a calibration process that minimizes the information loss when approximating the FP32 network with a limited 8-bit integer representation. Refer to this blog to learn more about Fast INT8 Inference for Autonomous Vehicles with TensorRT 3.
Useful Resources:
- Introduction to TensorRTÂ (webinar)
- TensorRT Best Practices guide
- TensorRT 4 Overview
- 8-Bit Inference with TensorRTÂ
- Optimizing NMT with TensorRT
If you have issues with TensorRT, check the NVIDIA TensorRT Developer Forum to see if others members of the TensorRT community have a resolution first. NVIDIA Registered Developer Program can also file bugs at https://developer.nvidia.com/nvidia-developer-program.
References
1CPU: Skylake Gold 6140, 2.3GHz 3.7 GHz Turbo; HT On; 2 sockets enabled, 72 CPU threads enabled. Ubuntu 16.04 (OS Kernel Ver 4.13.0-39-generic); GPU: Tesla T4; CUDA (r410.72, version 10.0.145); TensorRT 5.0.2.1; Batch size: CPU=1; ECC Off.