A team of researchers from Princeton and the University of Washington created a new camera that captures stunning images and measures in at only a half-millimeter—the size of a coarse grain of salt.
The new study, published in Nature Communications, outlines the use of optical metasurfaces with machine learning to produce high-quality color imagery, with a wide field of view. The device could be used across industries ranging from robotics to most notably the medical field, to help with disease diagnosis.
Optical metasurfaces rely on a new method of light manipulation, using cylindrical posts set on a small, square surface. The posts, which vary in geometry, work like antennas that can capture incoming photons (waves of electromagnetic radiation). These waves are then sent as signals from the metasurface to a computer to interpret and produce an image.
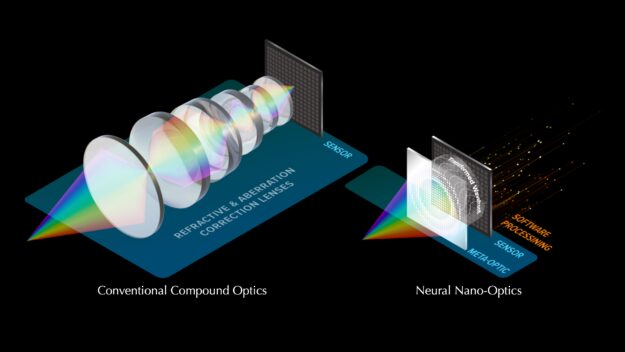
Tiny cameras offer vast potential for medical use, from brain imaging to minimally invasive endoscopies. But, up to this point, the technology has captured blurry, distorted images, with a limited field of view.
The researchers employed neural nano-optics—which combines optical metasurfaces with neural feature-based image reconstruction—to interpret the data and produce higher-quality images.
“To recover images from the measured data, we propose a feature-based deconvolution method that employs a differentiable inverse filter together with neural networks for feature extraction and refinement,” said senior author Felix Heide, an assistant professor of computer science at Princeton.
The team tested machine-learning algorithms with a simulator, comparing different configurations of the antennas. With 1.6 million cylinders on the optical surface and complex light interactions, the simulations demand massive amounts of memory.
The algorithm was tested and trained using the cuDNN-accelerated TensorFlow deep learning framework with an NVIDIA P100 GPU. The result is a new system capable of producing images comparable to a modern camera lens, from a device 500,000 times smaller. According to the study, the new camera is also 10 times better at filtering out errors than previous techniques.
“It’s been a challenge to design and configure these little microstructures to do what you want,” Ethan Tseng, a computer science Ph.D. student at Princeton and study co-lead said in a press release. “For this specific task of capturing large field of view RGB images, it’s challenging because there are millions of these little microstructures, and it’s not clear how to design them in an optimal way.”
The team is now working to add more computational abilities to the camera and envisions features such as object detection and sensors for medicine or robotics in future iterations. Beyond that, they see a use case where ultra-compact imagers turn surfaces into sensors.
“We could turn individual surfaces into cameras that have ultra-high resolution. So you wouldn’t need three cameras on the back of your phone anymore, but the whole back of your phone would become one giant camera. We can think of completely different ways to build devices in the future,” said Heide.
The raw capture data and code used to design and evaluate the neural nano-optic is publicly accessible. More information is also available on the Neural Nano-Optics for High-quality Thin Lens Imaging webpage.
Read the published research in Nature Communications. >>
Read the press release. >>
Feature image courtesy of Princeton/Ethan Tseng, et al.