Rapid digital transformation has led to an explosion of sensitive data being generated across the enterprise. That data has to be stored and processed in data centers on-premises, in the cloud, or at the edge. Examples of activities that generate sensitive and personally identifiable information (PII) include credit card transactions, medical imaging or other diagnostic tests, insurance claims, and loan applications.
This wealth of data presents an opportunity for enterprises to extract actionable insights, unlock new revenue streams, and improve the customer experience. Harnessing the power of AI enables a competitive edge in today’s data-driven business landscape.
However, the complex and evolving nature of global data protection and privacy laws can pose significant barriers to organizations seeking to derive value from AI:
- General Data Protection Regulation (GDPR) in Europe
- Health Insurance Portability and Accountability Act (HIPAA) and Gramm-Leach-Bliley Act (GLBA) in the United States
Customers in healthcare, financial services, and the public sector must adhere to a multitude of regulatory frameworks and also risk incurring severe financial losses associated with data breaches.

In addition to data, the AI models themselves are valuable intellectual property (IP). They are the result of significant resources invested by the model owners in building, training, and optimizing. When not adequately protected in use, AI models face the risk of exposing sensitive customer data, being manipulated, or being reverse-engineered. This can lead to incorrect results, loss of intellectual property, erosion of customer trust, and potential legal repercussions.
Data and AI IP are typically safeguarded through encryption and secure protocols when at rest (storage) or in transit over a network (transmission). But during use, such as when they are processed and executed, they become vulnerable to potential breaches due to unauthorized access or runtime attacks.
Preventing unauthorized access and data breaches
Confidential computing addresses this gap of protecting data and applications in use by performing computations within a secure and isolated environment within a computer’s processor, also known as a trusted execution environment (TEE).
The TEE acts like a locked box that safeguards the data and code within the processor from unauthorized access or tampering and proves that no one can view or manipulate it. This provides an added layer of security for organizations that must process sensitive data or IP.
The TEE blocks access to the data and code, from the hypervisor, host OS, infrastructure owners such as cloud providers, or anyone with physical access to the servers. Confidential computing reduces the surface area of attacks from internal and external threats. It secures data and IP at the lowest layer of the computing stack and provides the technical assurance that the hardware and the firmware used for computing are trustworthy.
Today’s CPU-only confidential computing solutions are not sufficient for AI workloads that demand acceleration for faster time-to-solution, better user experience, and real-time response times. Extending the TEE of CPUs to NVIDIA GPUs can significantly enhance the performance of confidential computing for AI, enabling faster and more efficient processing of sensitive data while maintaining strong security measures.
Secure AI on NVIDIA H100
Confidential computing is a built-in hardware-based security feature introduced in the NVIDIA H100 Tensor Core GPU that enables customers in regulated industries like healthcare, finance, and the public sector to protect the confidentiality and integrity of sensitive data and AI models in use.
With security from the lowest level of the computing stack down to the GPU architecture itself, you can build and deploy AI applications using NVIDIA H100 GPUs on-premises, in the cloud, or at the edge. No unauthorized entities can view or modify the data and AI application during execution. This protects both sensitive customer data and AI intellectual property.
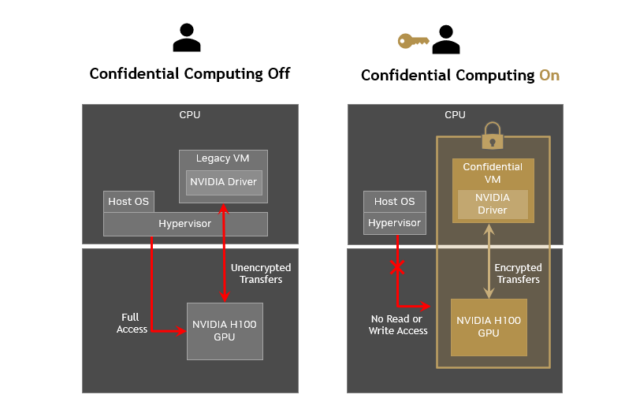
For a quick overview of the confidential computing feature in NVIDIA H100, see the following video.
Unlocking secure AI: Use cases empowered by confidential computing on NVIDIA H100
Accelerated confidential computing with NVIDIA H100 GPUs offers enterprises a solution that is performant, versatile, scalable, and secure for AI workloads. It unlocks new possibilities to innovate with AI while maintaining security, privacy, and regulatory compliance.
- Confidential AI training
- Confidential AI inference
- AI IP protection for ISVs and enterprises
- Confidential federated learning
Confidential AI training
In industries like healthcare, financial services, and the public sector, the data used for AI model training is sensitive and regulated. This includes PII, personal health information (PHI), and confidential proprietary data, all of which must be protected from unauthorized internal or external access during the training process.
With confidential computing on NVIDIA H100 GPUs, you get the computational power required to accelerate the time to train and the technical assurance that the confidentiality and integrity of your data and AI models are protected.
For AI training workloads done on-premises within your data center, confidential computing can protect the training data and AI models from viewing or modification by malicious insiders or any inter-organizational unauthorized personnel. When you are training AI models in a hosted or shared infrastructure like the public cloud, access to the data and AI models is blocked from the host OS and hypervisor. This includes server administrators who typically have access to the physical servers managed by the platform provider.
Confidential AI inference
When trained, AI models are integrated within enterprise or end-user applications and deployed on production IT systems—on-premises, in the cloud, or at the edge—to infer things about new user data.
End-user inputs provided to the deployed AI model can often be private or confidential information, which must be protected for privacy or regulatory compliance reasons and to prevent any data leaks or breaches.
The AI models themselves are valuable IP developed by the owner of the AI-enabled products or services. They are at risk of being viewed, modified, or stolen during inference computations, resulting in incorrect results and loss of business value.
Deploying AI-enabled applications on NVIDIA H100 GPUs with confidential computing provides the technical assurance that both the customer input data and AI models are protected from being viewed or modified during inference. This provides an added layer of trust for end users to adopt and use the AI-enabled service and also assures enterprises that their valuable AI models are protected during use.
AI IP protection for ISVs and enterprises
Independent software vendors (ISVs) invest heavily in developing proprietary AI models for a variety of application-specific or industry-specific use cases. Examples include fraud detection and risk management in financial services or disease diagnosis and personalized treatment planning in healthcare.
ISVs must protect their IP from tampering or stealing when it is deployed in customer data centers on-premises, in remote locations at the edge, or within a customer’s public cloud tenancy. In addition, customers need the assurance that the data they provide as input to the ISV application cannot be viewed or tampered with during use.

Confidential computing on NVIDIA H100 GPUs enables ISVs to scale customer deployments from cloud to edge while protecting their valuable IP from unauthorized access or modifications, even from someone with physical access to the deployment infrastructure. ISVs can also provide customers with the technical assurance that the application can’t view or modify their data, increasing trust and reducing the risk for customers using the third-party ISV application.
Confidential federated learning
Building and improving AI models for use cases like fraud detection, medical imaging, and drug development requires diverse, carefully labeled datasets for training. This demands collaboration between multiple data owners without compromising the confidentiality and integrity of the individual data sources.
Confidential computing on NVIDIA H100 GPUs unlocks secure multi-party computing use cases like confidential federated learning. Federated learning enables multiple organizations to work together to train or evaluate AI models without having to share each group’s proprietary datasets.
Confidential federated learning with NVIDIA H100 provides an added layer of security that ensures that both data and the local AI models are protected from unauthorized access at each participating site.
When deployed at the federated servers, it also protects the global AI model during aggregation and provides an additional layer of technical assurance that the aggregated model is protected from unauthorized access or modification.
This helps drive the advancement of medical research, expedite drug development, mitigate insurance fraud, and trace money laundering globally while maintaining security, privacy, and regulatory compliance for all parties involved.
NVIDIA platforms for accelerated confidential computing on-premises
Getting started with confidential computing on NVIDIA H100 GPUs requires a CPU that supports a virtual machine (VM)–based TEE technology, such as AMD SEV-SNP and Intel TDX. Extending the VM-based TEE from the supported CPU to the H100 GPU enables all the VM memory to be encrypted and the application running does not require any code changes.
Top OEM partners are now shipping accelerated platforms for confidential computing, powered by NVIDIA H100 Tensor Core GPUs. These confidential computing–compatible systems combine the NVIDIA H100 PCIe Tensor Core GPUs with AMD Milan or AMD Genoa CPUs that support the AMD SEV-SNP technology.
The following partners are delivering the first wave of NVIDIA platforms for enterprises to secure their data, AI models, and applications in use in data centers on-premises:
- ASRock Rack
- ASUS
- Cisco
- Dell Technologies
- GIGABYTE
- Hewlett Packard Enterprise
- Lenovo
- Supermicro
- Tyan
NVIDIA H100 GPU comes with the VBIOS (firmware) that supports all confidential computing features in the first production release. The NVIDIA confidential computing software stack to be released this summer will support single GPU first and then multi-GPU and Multi-Instance GPU in subsequent releases.
For more information about the NVIDIA confidential computing software stack and availability, see the Unlock the Potential of AI with Confidential Computing on NVIDIA GPUs session (Track 2) at the Confidential Computing Summit 2023 on June 29.