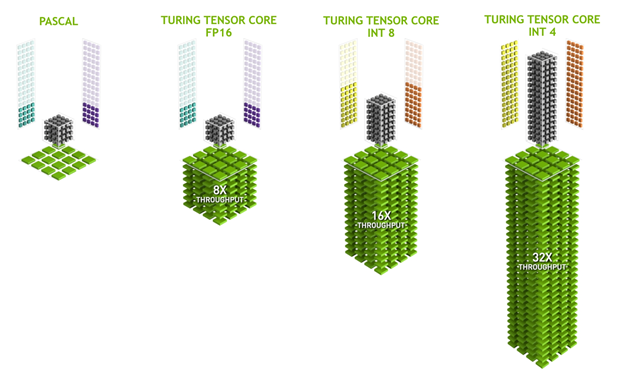
Every year, clever researchers introduce ever more complex and interesting deep learning models to the world. There is of course a big difference between a model that works as a nice demo in isolation and a model that performs a function within a production pipeline.
This is particularly pertinent to creative apps where generative models must run with low latency to generate or enhance image– or video-based content.
In many situations, to reduce latency and provide the best interaction, you often want to perform inference on a local workstation GPU rather than the cloud.
There are several constraints to consider when deploying to the workstation:
- Hardware
- This is unknown when you build the model.
- This may change after installation. A user may have a GTX1060 one day and an RTX6000 the next.
- Resources
- When they’re deployed in the cloud, resources are a lot more predictable than when they’re deployed on a workstation.
The overriding advantage of workstation execution is the removal of any extra latency going to and from a remote service that may not already be guaranteed.
NVIDIA Tensor Cores
On NVIDIA RTX hardware, from the Volta architecture forward, the GPU includes Tensor Cores to enable acceleration of some of the heavy lift operations involved with deep learning. Essentially, the Tensor Cores enable an operation called warp matrix multiply-accumulate (wmma), providing optimized paths for FP16-based (hmma) and integer-based (imma) matrix multiplication.
To take full advantage of the hardware acceleration, it’s important to understand the exact capabilities of the Tensor Cores.
Convolutional neural networks contain many convolution layers that, when you examine the core operation, come down to many dot products. These operations can be batched together to run as a single, large, matrix multiplication operation.
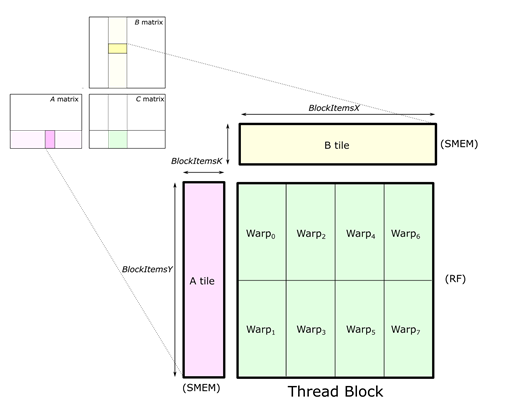
The acceleration of large matrix multiplications is something that GPUs do very well if they use optimal memory access patterns, which can be implemented using libraries such as CUTLASS. Tensor Cores provide the operation with a boost at the most crucial part of the operation, when the per-block dot products are accumulated.
WinML and Tensor Cores
Models that run on Windows Machine Learning (WinML) using ONNX can benefit from Tensor Cores on NVIDIA hardware, but it is not immediately obvious how to make sure that they are in fact used. There is no switch or button labeled Use Tensor Cores and there are certain constraints by which the model and input data must abide.
Figure 3 shows how Microsoft has structured WinML.
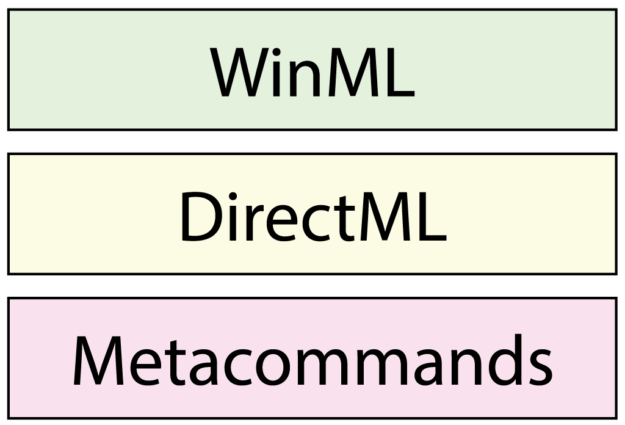
- WinML—Consumes ONNX models and under the hood runs a few high level optimization passes to generate a sequence of DirectML commands to run the model.
- DirectML—Allows you to implement models directly. Uses DirectX compute to run operations.
- Metacommands—Mechanism by which independent hardware providers (such as NVIDIA) can implement overridden versions of operations making the best use of the hardware
When a WinML model is evaluated and hits, for example, a convolution that would be mapped to a DirectML command, the runtime first looks for a metacommand. a metacommand likely exists as long as the constraints for them are satisfied.
The metacommand analyzes the input and parameters pertaining to the command and makes sure that the constraints for running WMMA are satisfied. If they are, a set of kernels that make use of Tensor Cores is selected for the operation. If they are not satisfied, or no Tensor Cores are available, the metacommand falls back to a different approach.
Precision, precision, precision…
To maximize the throughput and keep all the respective units busy, there is a constraint when working with floating point operations that the input to the Tensor Core be FP16. The A and B operands of the matrix are multiplied together to produce either FP16 or FP32 output. In the latter case, where you produce a 32-bit output, there is a performance penalty. You end up running the operation at half the speed that you could be, if you did not mix precision.
While it is possible to get other APIs such as cuDNN to consume FP32 into a Tensor Core operation, all that this is really doing is reducing the precision of the input immediately before the Tensor Core operation. In contrast, when you use WinML and ONNX, the input to the model and the model parameters (weights) must be FP16.
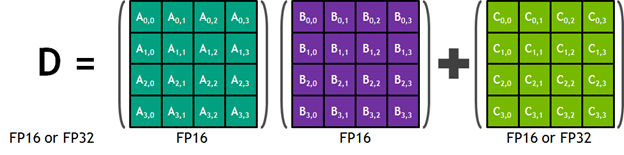
It may be tempting to assume that a lower precision can mean a lower quality output. But this is rarely the case, particularly when dealing with images and video in a standard dynamic range. FP16 gives you around 4x the precision of 8-bit UINT, anyway. Typically, the variance of most models is in the -1 to 1 range.
Producing a model that has FP16 weights is something that most, if not all conversion tools do for you. You still need to provide the input as FP16, so what is the best way to do this? There are several options available:
- Convert on the CPU and copy a smaller amount of data to the GPU:
- While this might seem like a good option because you have less data to copy, consider the fact that reducing the precision of a large amount of data is still time-consuming, certainly more so than the copy.
- If your data is already on the GPU but in UINT8 or FP32, you’d incur even more overhead in copying back to the CPU, performing operations such as conversion to FP16 and pre/post processing, then copying back to the GPU again.
- Convert to FP16 on the GPU using WinML’s custom operator provider:
- This method allows you to leverage the GPU’s parallelism to convert the data to FP16.
- It also enables you to fuse this operation with common pre-processing operations such as normalization or mean subtraction.
Generally speaking, you can improve performance considerably if you do not mix precision. Mixed precision is in most cases supported, but the metacommand must perform extra work to make sure that everything works as expected. This usually means changing the precision of data in the model at runtime so that everything matches up.
Custom operators in WinML
At first glance, WinML and ONNX might seem like a bit of a black box. However, a set of interfaces exists that allows you to implement your own custom operators and provide the necessary hooks into ONNX to run them.
When I use the term operator in the context of a deep learning model, I’m referring to an operation such as a 2D convolution or activation. By custom operator, I mean an operation that is not defined as part of the standard implementation of an API or framework but one that you define.
ONNX considerations
To maintain compatibility in the ever-evolving field of deep learning operators, ONNX models maintain what is known as an operator set (opset) version. There can be a version disparity in opset support between ONNX and WinML. For example, at the time of publication, ONNX is at version 11 and WinML at version 8. This seems like a problem; however, you can import your own operator set to sit along the standard ONNX opset and then infer against your model.
Operators and opsets exist within a domain, which acts very much like a namespace. Operator names must be unique within a given domain. As WinML can consume ONNX models with more than one operator set, it is possible to create new operators to do computations that the default opset cannot handle. You can also create new operators that override the defaults, by pointing the operator at a different domain.
WinML considerations
When you set up the WinML environment and consume a model, you can do so by using the method in the following code example:
m_model = LearningModel::LoadFromFilePath(m_model_path,[m_custom_op_provider]);
The second parameter is optional and allows you to pass in a custom operator provider to service bespoke operations. For more information, see the samples available from Microsoft that cover the creation of custom operators.
Custom operators are a key tool to avoid CPU round trips and allow optimized load and store behavior on the GPU.
Depending on the amount of required preprocessing operations, shared memory and registers should be used effectively to maximize the number of math operations per global load store (that is, maintain a high compute to memory access ratio).
At this point, I should point out that there are a few useful tools available from the Microsoft WinML GitHub repository:
- WinMLRunner—This tool is a simple WinML framework that consumes an ONNX file and dumps the output. This can be useful in evaluating models for performance and completeness before engaging in the task of setting up your own integration workflow.
- WinML Dashboard—This has a few useful functions. First, it provides a graphic view of a model’s architecture and provides limited graph editing capabilities. Second, it provides a utility to convert models to ONNX from other frameworks, such as PyTorch and TensorFlow. Lastly, it also provides a graphical UI around WinMLRunner, allowing you to evaluate models directly from the dashboard.
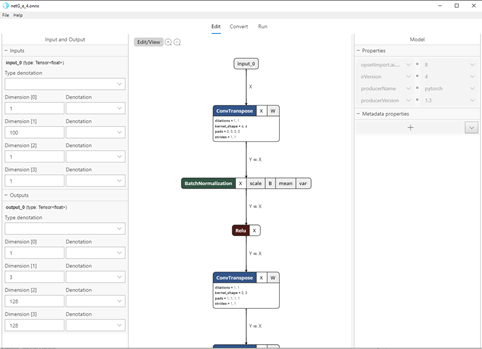
Other considerations
It is crucial for WinML to know the input and batch size for the model ahead of time so that Tensor Cores can be used. While it is possible for these values to be inferred from the input data itself, providing them explicitly enables opportunities for the runtime to optimize.
When you are performing linear operations, the batch size needs to be a multiple of 8 for HMMA (FP16) or 16 for IMMA (int). For the same reason, when you are performing a convolution operation, both the input and output channel filter counts need to be a multiple of 8 or 16 (for HMMA and IMMA, respectively).
To get best Tensor Core utilization and performance, try to keep the input dimensions in multiples of 64/128/256, and try to keep the dimensions as large as possible (within reason, given memory constraints). The operation is broken down into tiles of (for example) 16x8x8.
Make sure that there are enough tiles created to fully occupy all the compute units (SMs) on the target . When the input and output filter counts for convolutions are multiples of 32, however, the WinML metacommand can provide better utilization of the Tensor Cores and yield higher performance.
Data layout is another factor that affects performance considerably. When I present data to an operation, I usually provide it either in the NCHW layout (planar) or the NHWC layout (interleaved) . While the former may seem like it would map better to a deep learning problem, the latter yields better performance on Tensor Cores.
The reason for this also relates to why you must have multiples of eight input and output feature maps. Tensor Cores are very sensitive to memory bandwidth and are only effective if you can feed them fast enough. It is crucial to keep memory throughput to a maximum.
When you provide data in NCHW (planar) layout, there is poor spatial locality between channels. However, if you provide data in NHWC (Interleaved) layout, and batch eight channels together, you can make effective use of coalesced loads and reduce the number of memory transactions that are required to fill the units.
While the metacommand implementation has the ability to perform the necessary transposition, doing so of course incurs a performance penalty.
It’s important to pay attention to data layout when dealing with WinML. After the conversion of your model, it is well worth using a tool such as WinML Dashboard to see what kind of conversion has been done. If you see transpose nodes scattered across your model, consider addressing your architecture.
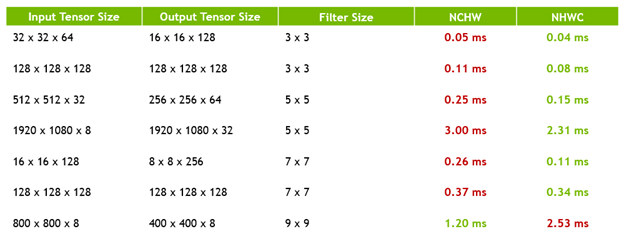
Performance
Taking these guidelines into consideration, what kind of speedup can you expect? As is usual in development, there can be a lot of factors, such as how your model is composed or how much of it can in fact be accelerated by Tensor Cores.
That said, in terms of the linear and convolution layers that exist, the maximum theoretical speedup is around 24x. In practice, a speedup of 16x to 20x can be considered good. Over a complete model, considering the many other operations that take place, an average large model hypothetically based on ResNet-50 would get an overall speedup of around 4x. But this is very much a rule of thumb, and these figures can vary .
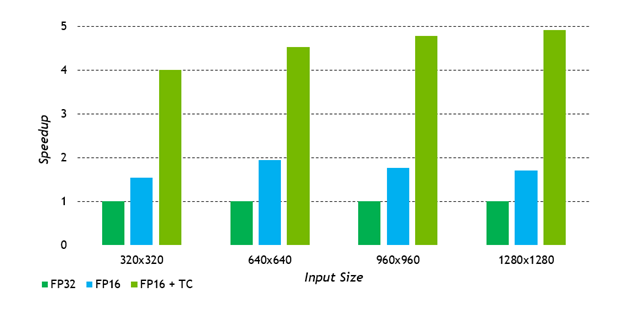
Another benefit of working with reduced precision is the reduced memory footprint. AI models can be large, even on the order of many GBs of network parameters. You can effectively halve the memory for both the runtime and storage footprints of a model by reducing to FP16 and halve that again by quantizing to UINT8.
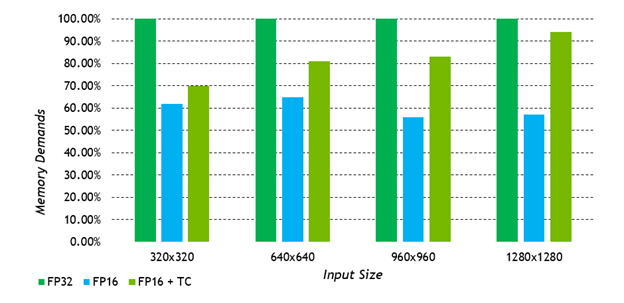
Conclusion
WinML is a very powerful tool but can be quite abstract. In some respects, this is both a blessing and a curse. On the one hand, WinML with ONNX provides a straightforward solution to move from research to production quickly. On the other hand, to achieve optimum performance, you must take care to make sure that ONNX files are well-generated.
Checklists are helpful when it comes to the production phase of any project. To leverage NVIDIA hardware effectively and make sure that Tensor Cores effectively execute a model using WinML, use the following checklist:
- Use FP16 for the model and the input.
- Avoid mixed precision.
- Fuse any format conversion with other operations, if you can.
- Fuse any format conversion with other operations, if you can.
- Stick to the NHWC layout. Precompute any necessary transposition into the model.
- Avoid transposes at runtime.
- Fully use the GPU.
- Make sure that input/output filter counts are at least a multiple of eight. Ideally, make them a multiple of 32 or more.
- Avoid transfers to and from the GPU or CPU.
- Use custom operators for any bespoke processing.