NVIDIA TensorRT
NVIDIA® TensorRT™, an SDK for high-performance deep learning inference, includes a deep learning inference optimizer and runtime that delivers low latency and high throughput for inference applications.
Download Now Get StartedNVIDIA TensorRT Benefits
Speed Up Inference by 36X
NVIDIA TensorRT-based applications perform up to 36X faster than CPU-only platforms during inference, enabling you to optimize neural network models trained on all major frameworks, calibrate for lower precision with high accuracy, and deploy to hyperscale data centers, embedded platforms, or automotive product platforms.
Optimize Inference Performance
TensorRT, built on the NVIDIA CUDA® parallel programming model, enables you to optimize inference using techniques such as quantization, layer and tensor fusion, kernel tuning, and others on NVIDIA GPUs.
Accelerate Every Workload
TensorRT provides INT8 using quantization-aware training and post-training quantization and floating point 16 (FP16) optimizations for deployment of deep learning inference applications, such as video streaming, recommendations, fraud detection, and natural language processing. Reduced-precision inference significantly minimizes latency, which is required for many real-time services, as well as autonomous and embedded applications.
Deploy, Run, and Scale With Triton
TensorRT-optimized models can be deployed, run, and scaled with NVIDIA Triton™, open-source, inference-serving software that includes TensorRT as one of its backends. The advantages of using Triton include high throughput with dynamic batching and concurrent model execution as well as features such as model ensembles, streaming audio/video inputs, and more.
Inference for Large Language Models
NVIDIA TensorRT-LLM
NVIDIA TensorRT-LLM is an open-source library that accelerates and optimizes inference performance of the latest large language models (LLMs) on the NVIDIA AI platform. It lets developers experiment with new LLMs, offering high performance and quick customization without requiring deep knowledge of C++ or CUDA.
Developers can now accelerate LLM performance on NVIDIA’s datacenter systems, to local desktop and laptop GPUs – including NVIDIA RTX Systems on native Windows – with the same seamless workflow.
TensorRT-LLM wraps TensorRT’s deep learning compiler—which includes optimized kernels from FasterTransformer, pre- and post-processing, and multi-GPU and multi-node communication—in a simple open-source Python API for defining, optimizing, and executing LLMs for inference in production.
Read Our Blog To Learn How You Can Get Started
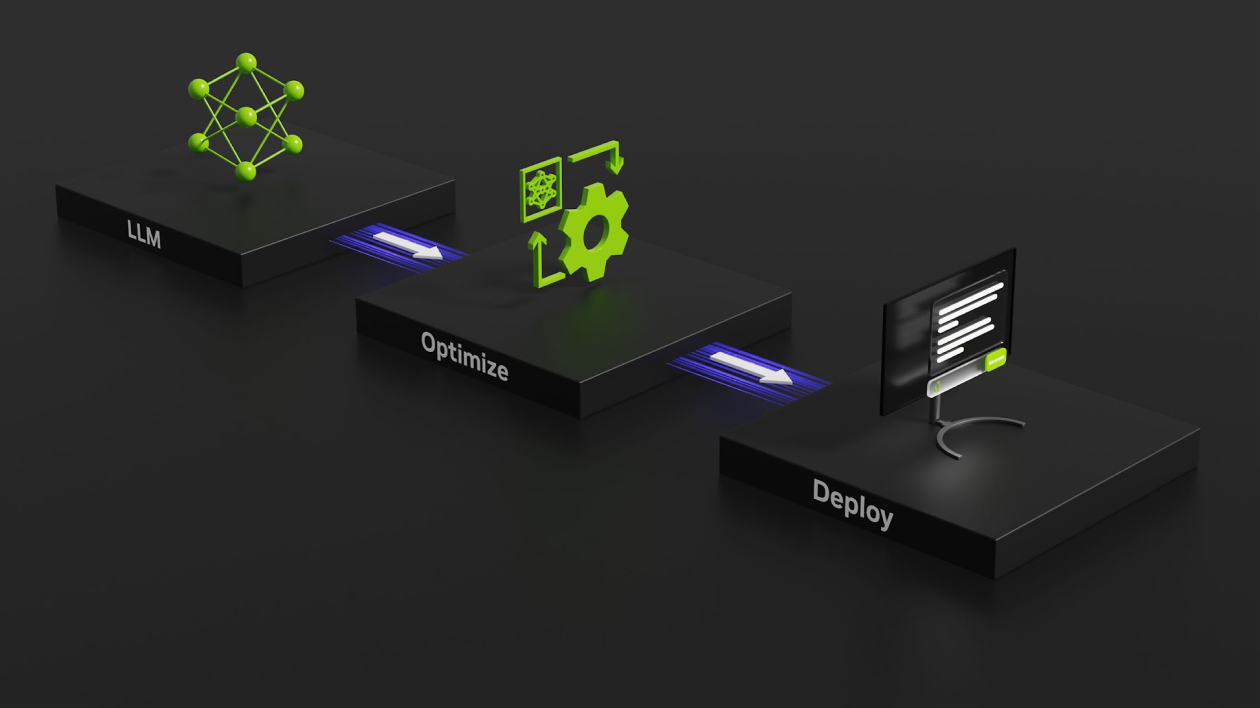
World-Leading Inference Performance
TensorRT was behind NVIDIA’s wins across all performance tests in the industry-standard benchmark for MLPerf Inference. TensorRT-LLM accelerates the latest large language models for generative AI, delivering up to 8X more performance, 5.3X better TCO, and nearly 6X lower energy consumption.
8X Increase in GPT-J 6B Inference Performance
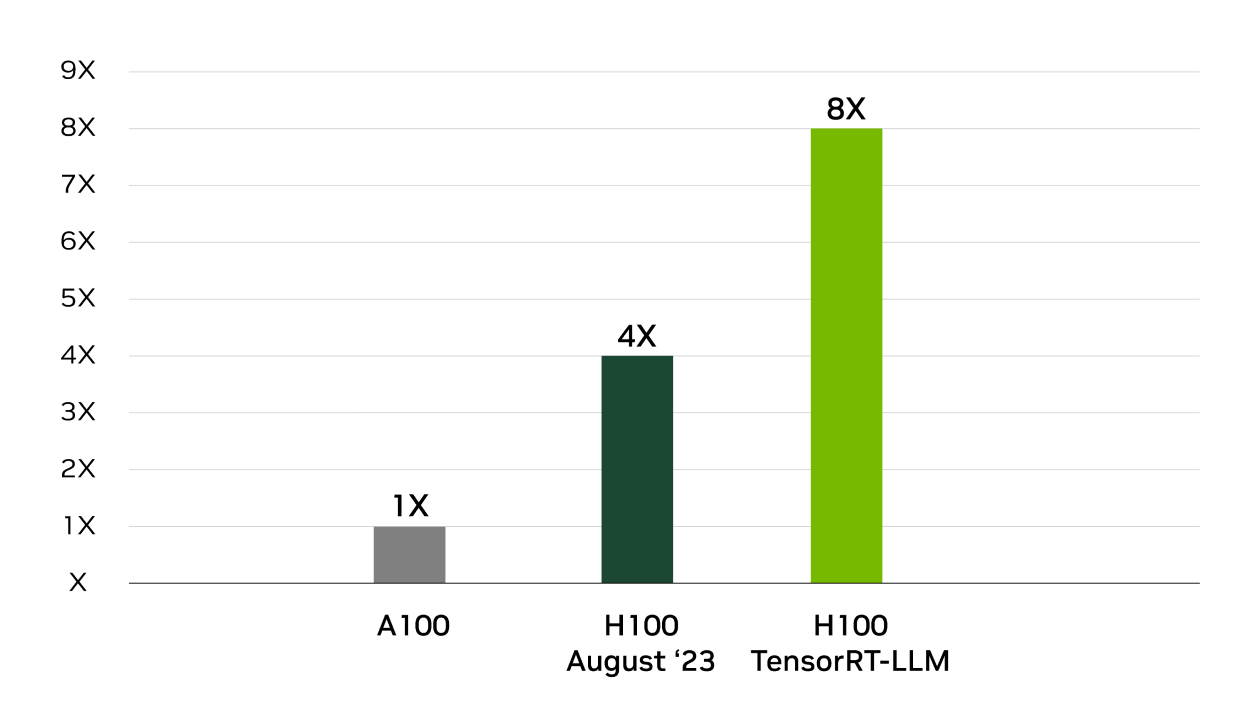
4X Higher Llama2 Inference Performance
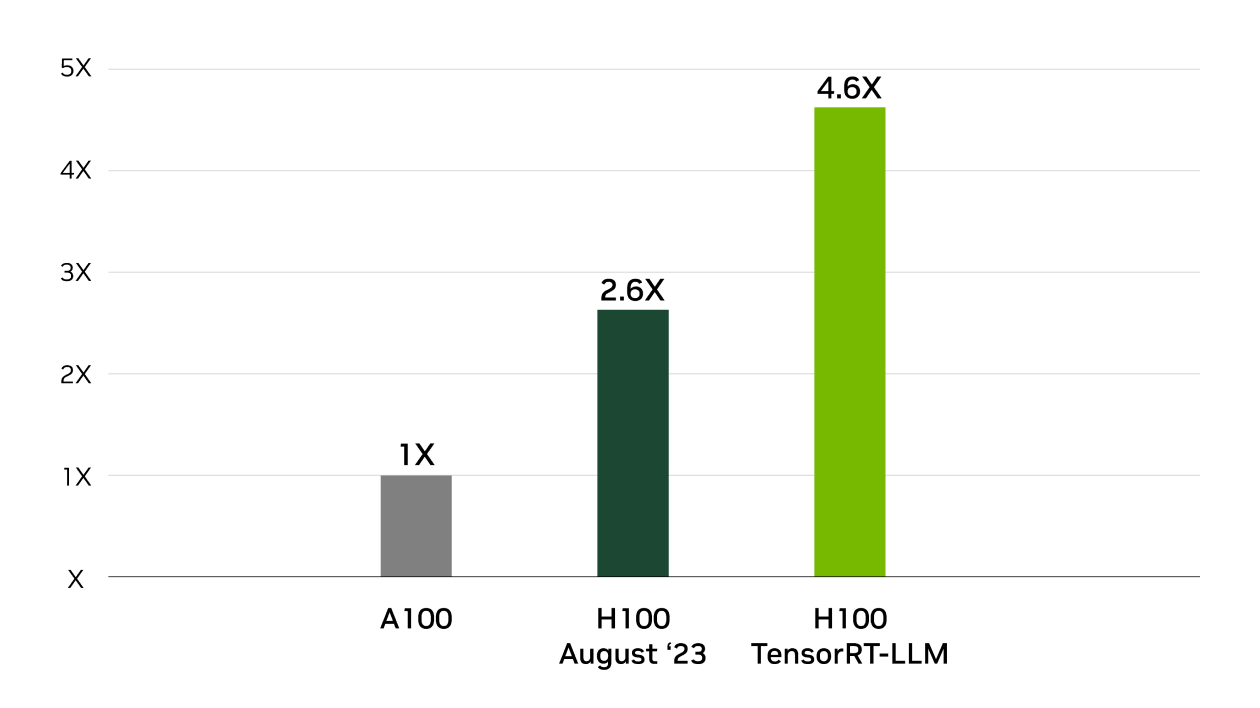
Total Cost of Ownership
Lower is better
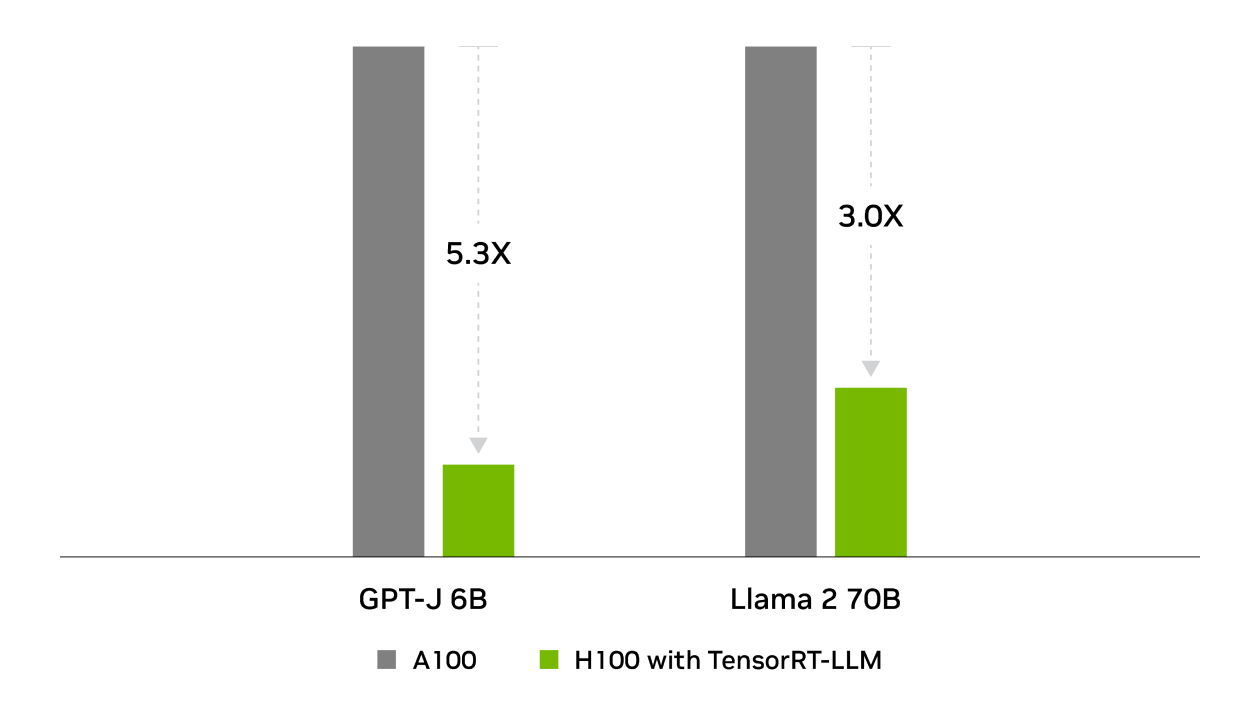
Energy Use
Lower is better
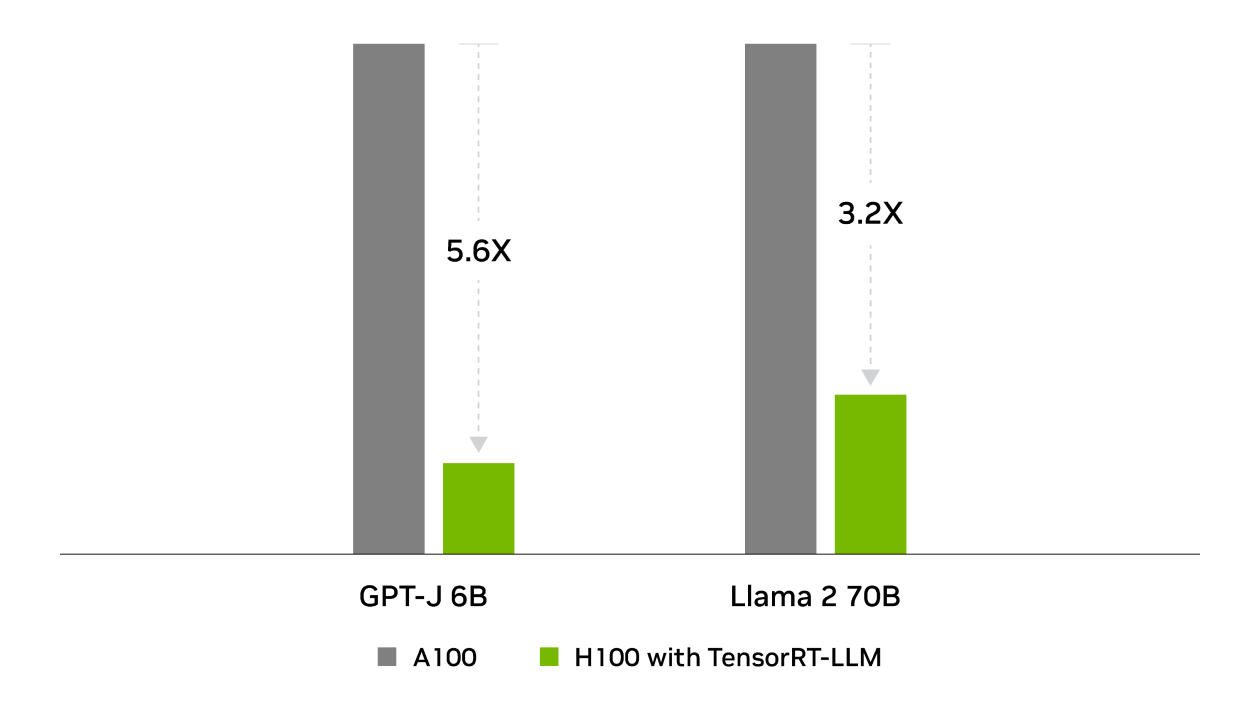
Ways to Get Started With NVIDIA TensorRT
Purchase NVIDIA AI Enterprise
Purchase NVIDIA AI Enterprise, an end-to-end AI software platform that includes TensorRT and TensorRT-LLM, for mission-critical AI inference with enterprise-grade security, stability, manageability and support.
Apply for a 90-Day NVIDIA AI Enterprise Evaluation License Contact Us to Learn More About Purchasing TensorRTDownload Containers, Code, and Releases
TensorRT is available as a binary on multiple different platforms, or as a container on NVIDIA NGC™. TensorRT is also integrated into the NGC containers for PyTorch, TensorFlow, and Triton Inference Server.
Download TensorRT Pull the TensorRT Container From NGC Access TensorRT-LLM Repository Explore More Resources for DevelopmentAccelerate Every Inference Platform
TensorRT can optimize and deploy applications to the data center, as well as embedded and automotive environments. It powers key NVIDIA solutions such as NVIDIA TAO, NVIDIA DRIVE™, NVIDIA Clara™, and NVIDIA Jetpack™.
TensorRT is also integrated with application-specific SDKs, such as NVIDIA DeepStream, NVIDIA Riva, NVIDIA Merlin™, NVIDIA Maxine™, NVIDIA Morpheus, and NVIDIA Broadcast Engine to provide developers with a unified path to deploy intelligent video analytics, speech AI, recommender systems, video conference, AI based cybersecurity, and streaming apps in production.
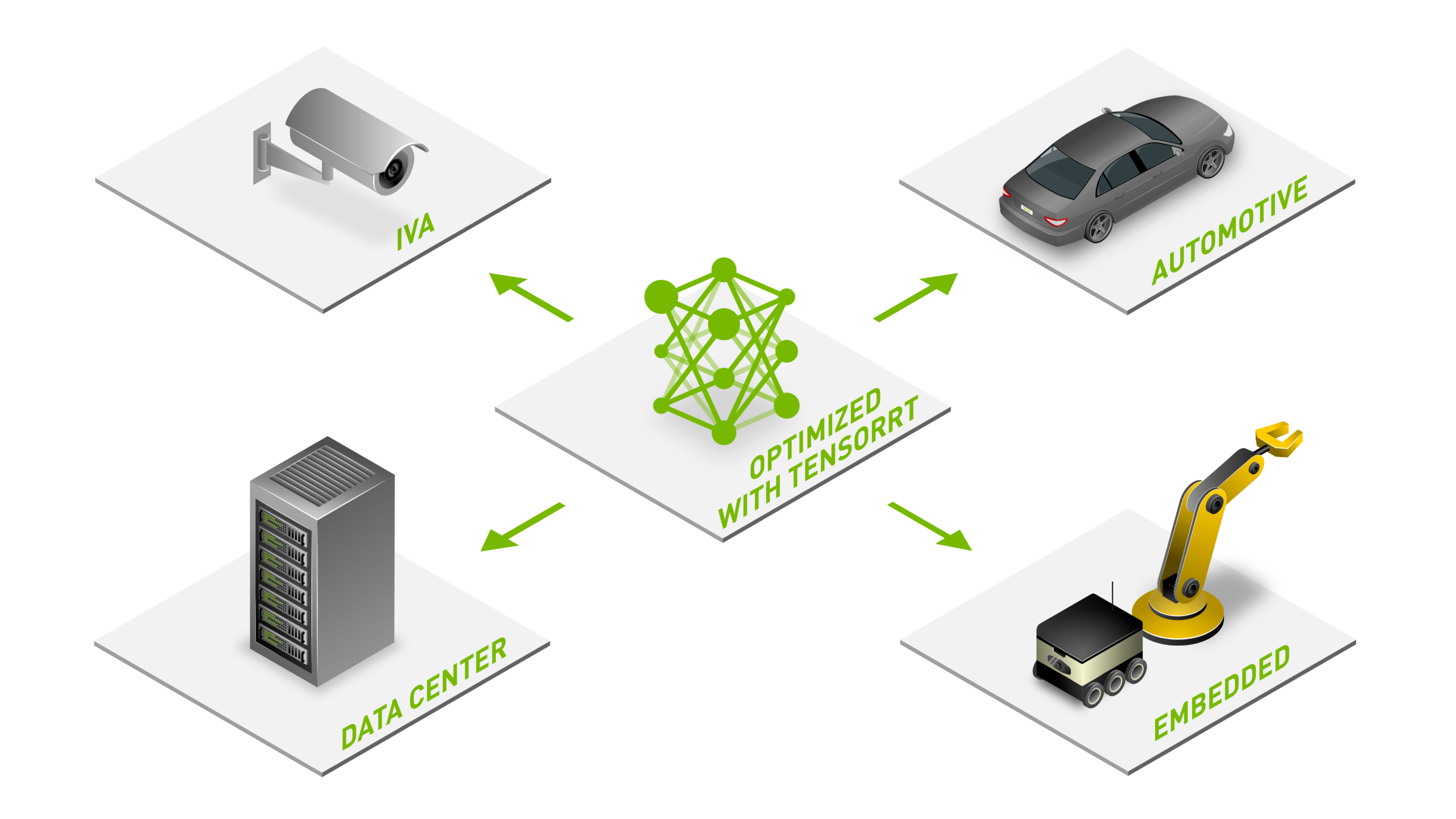
Supports Major Frameworks
TensorRT is integrated with PyTorch and TensorFlow so you can achieve 6X faster inference with a single line of code. If you’re performing deep learning training in a proprietary or custom framework, use the TensorRT C++ API to import and accelerate your models. Read more in the TensorRT documentation.
Below are a few integrations with information on how to get started.
PyTorch
Accelerate PyTorch models using the new Torch-TensorRT Integration with just one line of code. Get 6X faster inference using the TensorRT optimizations in a familiar PyTorch environment.
Learn MoreTensorFlow
TensorRT and TensorFlow are tightly integrated so you get the flexibility of TensorFlow with the powerful optimizations of TensorRT like 6X the performance with one line of code.
Learn MoreONNX
TensorRT provides an ONNX parser so you can easily import ONNX models from popular frameworks into TensorRT. It’s also integrated with ONNX Runtime, providing an easy way to achieve high-performance inference in the ONNX format.
Learn MoreMatlab
MATLAB is integrated with TensorRT through GPU Coder so you can automatically generate high-performance inference engines for NVIDIA Jetson™, NVIDIA DRIVE®, and data center platforms.
Learn MoreRead Success Stories
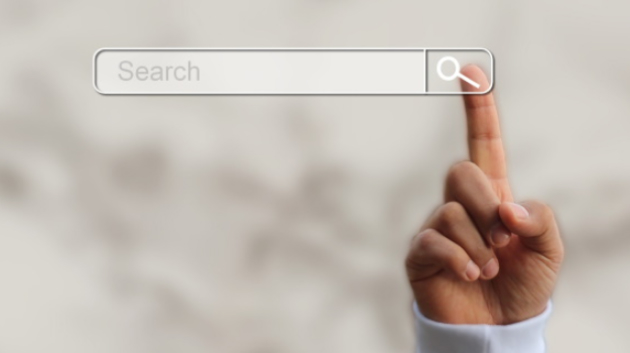
Discover how Amazon improved customer satisfaction by accelerating its inference 5X faster.
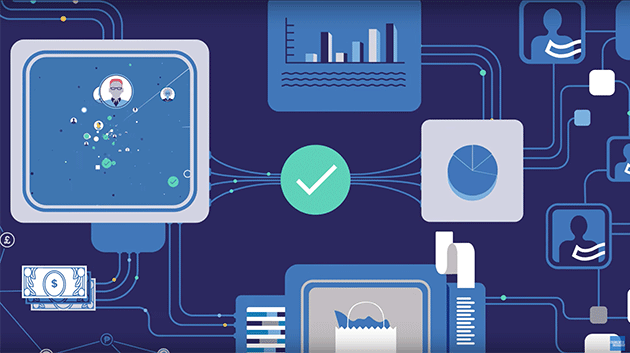
American Express improves fraud detection by analyzing tens of millions of daily transactions 50X faster. Find out how.
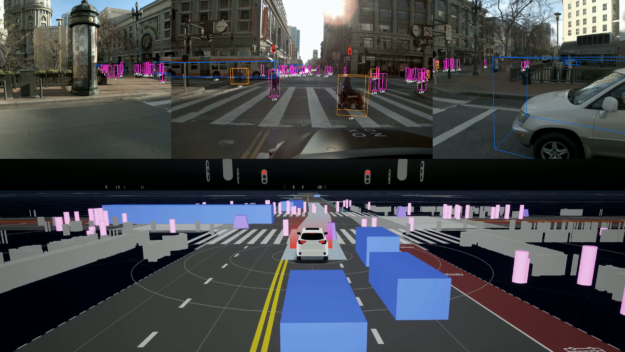
Explore how Zoox, a robotaxi startup, accelerated their perception stack by 19X using TensorRT for real-time inference on autonomous vehicles.
Widely-Adopted Across Industries
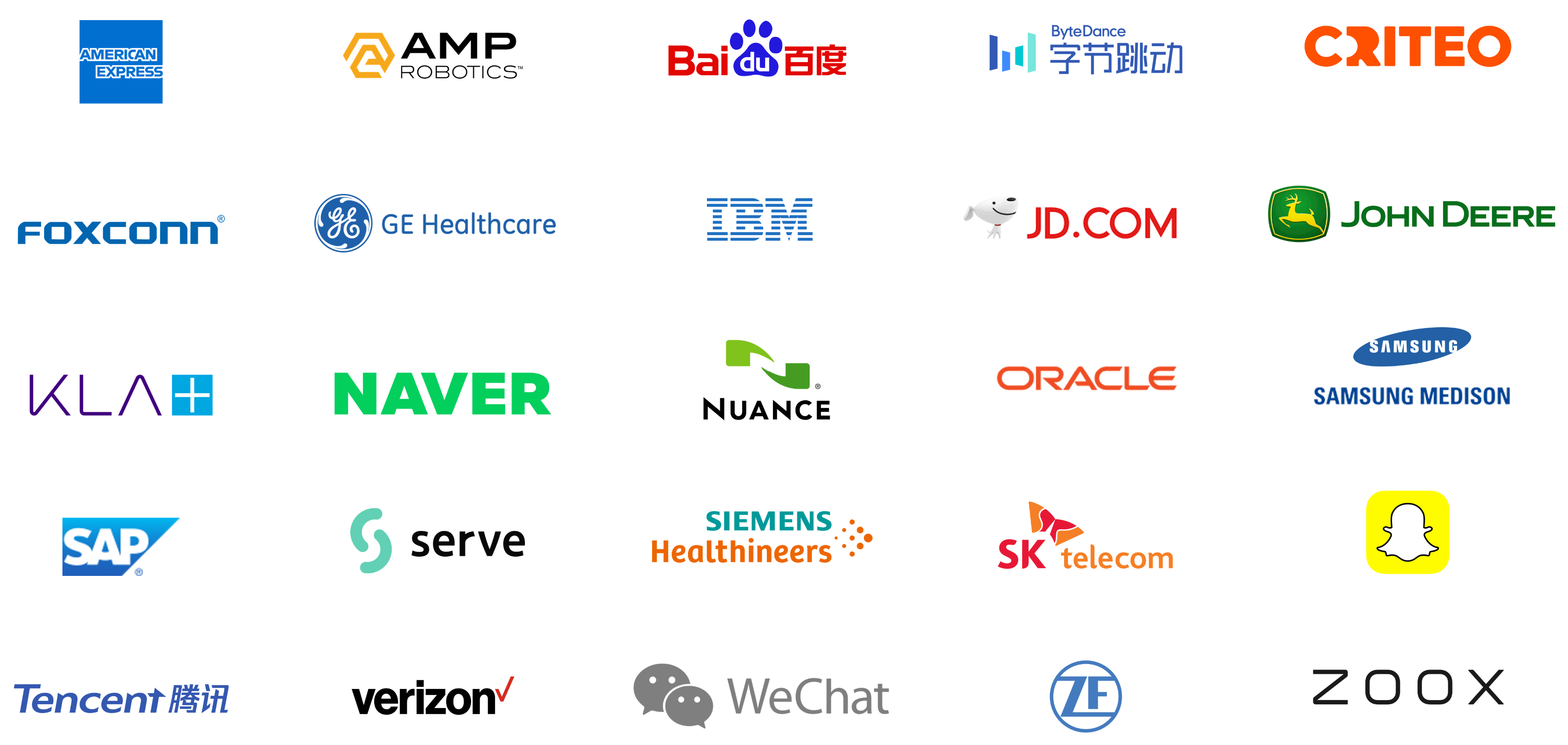
TensorRT Resources
Read the Introductory TensorRT Blog
Learn how to apply TensorRT optimizations and deploy a PyTorch model to GPUs.
Watch On-Demand TensorRT Sessions From GTC
Learn more about TensorRT and its new features from a curated list of webinars of GTC.
Get the Introductory Developer Guide
See how to get started with TensorRT in this step-by-step developer and API reference guide.
Stay up to date on the latest inference news from NVIDIA.