Because of climate change, vegetation zones in Alaska and the pan-arctic are shifting. This shift could affect ecosystem factors such as carbon cycling, permafrost dynamics, and fire regimes.
To help generate up-to-date vegetation maps that account for these shits, a team of scientists from Oak Ridge National Laboratory developed a deep learning-based method that can automatically generate high-resolution maps of vegetation in the region with 95% accuracy.
“Accurate vegetation maps are important for understanding terrestrial biosphere processes in high latitude ecosystems,” the researchers stated in their paper. “Given the importance of vegetation dynamics in Alaska and the associated impacts of climate change, there is a need for up-to-date and high-resolution vegetation maps.”
Using NVIDIA GeForce GPUs with the cuDNN-accelerated TensorFlow deep learning framework, the team trained a pair of convolutional neural networks on satellite imagery, synthetic radar data, topography, and other training data to classify vegetation. The end result was a fully-labeled vegetation map.
“We used unsupervised classification techniques with the ORNL-developed Mapcurves scheme to label the types of vegetation,” said ORNL’s Forrest Hoffman. “Then, we trained a deep neural network to produce a new map of vegetation communities.”
The scientists said they produced one of the most accurate, high resolution, field-validated vegetation maps for the Seward Peninsula of Alaska.
The team says they will scale the algorithms developed on the GeForce GPUs to an NVIDIA DGX workstation. From there the team plans to use the GPU-accelerated Summit supercomputer, the world’s fastest supercomputer, to generate much larger maps of the state of Alaska and the pan-Arctic.
A paper describing the method was recently published in the Remote Sensing Journal.
Read more>
ORNL Researchers Use AI to Map Arctic Vegetation
Feb 05, 2019
Discuss (0)
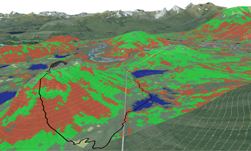
Related resources
- GTC session: Unlocking Secrets in Extreme Environments: An AIoT Platform for Long-Term and Real-Time Environmental Monitoring in Antarctica
- GTC session: Transforming 2D Imagery into 3D Geospatial Tiles With Neural Radiance Fields
- GTC session: Tool Sets for the AI Age (Presented by DigitalOcean)
- NGC Containers: MATLAB
- SDK: IndeX - Amazon Web Services
- Webinar: Isaac Developer Meetup #2 - Build AI-Powered Robots with NVIDIA Isaac Replicator and NVIDIA TAO