NVIDIA Modulus
NVIDIA Modulus is an open-source framework for building, training, and fine-tuning Physics-ML models with a simple Python interface.
Modulus empowers engineers to construct AI surrogate models that combine physics-driven causality with simulation and observed data, enabling real-time predictions. With generative AI using diffusion models, you can enhance engineering simulations and generate higher-fidelity data for scalable, responsive designs. Modulus supports the creation of large-scale digital twin models across various physics domains, from computational fluid dynamics and structural mechanics to electromagnetics.
Use Modulus to bolster your engineering simulations with AI. You can build models for enterprise-scale digital twin applications across multiple physics domains, from CFD and structural to electromagnetics.
Physics-Informed Machine Learning for Surrogate Models
Benefits
Modulus is an open-source, freely available AI framework for developing physics-ML models and novel AI architectures for engineering systems.
AI Toolkit for Physics
Quickly configure, build, and train AI models for physical systems in any domain, from engineering simulations to life sciences, with simple Python APIs.
Customize Models
Download, build on, and customize state-of-the-art pretrained models from the NVIDIA NGC™ catalog.
Near-Real-Time Inference
Deploy AI surrogate models as digital twins of your physical systems to simulate in near real time.
Scale With NVIDIA AI
Leverage NVIDIA AI to scale training performance from a single GPU to multi-node implementations.
Open-Source Design
Experience the benefits of open source. Modulus is built on top of PyTorch and is released under the Apache 2.0 license.
Standardized
Work with the best practices of AI development for physics-ML models, with an immediate focus on engineering applications.
User Friendly
Boost productivity with user-comprehensible error messages and easy-to-program Pythonic API interfaces.
High Quality
Use high-quality software with enterprise-grade development, tutorials for getting started, and robust validation and documentation.
See Modulus in Action
Speed and Accuracy of Gen AI Helps Combat Climate Change
Accelerating Extreme Weather Prediction with FourCastNet
Siemens Energy HRSG Digital Twin Simulation Using NVIDIA Modulus and Omniverse
Maximizing Wind Energy Production Using Wake Optimization
Accelerating Carbon Capture and Storage with Fourier Neural Operator and NVIDIA Modulus
Predicting Extreme Weather Events Three Weeks in Advance with FourCastNet
Contribute to Modulus’ Development
Modulus provides a unique platform for collaboration within the scientific community. Domain experts are invited to contribute and accelerate physics-ML across a variety of use cases and applications.
Key Features
New Model Architectures
Modulus offers a variety of approaches for training physics-based models, from purely physics-driven models like PINNs to physics-based, data-driven architectures such as neural operators, GNNs, and generative AI based diffusion models.
Modulus includes curated Physics-ML model architectures, Fourier feature networks, Fourier neural operators, GNNs, and diffusion models trained on NVIDIA DGX across open-source, free datasets found in the documentation.Training State-of-the-Art Physics-ML Models
Modulus provides an end-to-end pipeline for training Physics-ML models—from ingesting geometry to adding PDEs and scaling the training to multi-node GPUs. Modulus also includes training recipes in the form of reference applications.
DocumentationExplicit Parameterization
Modulus provides explicit parameter specifications for training the surrogate model with a range of values to learn for the design space and for inferring multiple scenarios simultaneously.
Omniverse Integration
Modulus is now integrated with the NVIDIA Omniverse™ platform for connecting and building custom 3D pipelines via an extension that can be used to visualize the outputs of a Modulus-trained model. The Modulus extension enables you to import the output results into a visualization pipeline for common output scenarios, such as streamlines and iso-surfaces. It also provides an interface that enables interactive exploration of design variables and parameters for inferring new system behavior and visualizing it in near real time.
Omniverse Integration Documentation
Production-Ready Solution With NVIDIA AI Enterprise
Modulus is now available with NVIDIA AI Enterprise, an end-to-end AI software platform optimized to accelerate enterprises to the leading edge of AI. NVIDIA AI Enterprise delivers validation and integration for NVIDIA AI open-source software, access to AI solution workflows to speed time to production, certifications to deploy AI everywhere, and enterprise-grade support, security, and API stability while mitigating the potential risks of open-source software
Learn more about NVIDIA AI Enterprise
Ways to Get Started With NVIDIA Modulus
Download Containers and Models for Development
Develop Physics-ML models using Modulus container and pretrained models, available for free on NVIDIA NGC.
Enterprise-Scale Workflows
Get free access to NVIDIA cloud workflows for Modulus and experience the ease of scaling to enterprise workloads.
Self-Paced Online Course
Take a hands-on introductory course from the NVIDIA Deep Learning Institute (DLI) to explore physics-informed machine learning with Modulus.
What Others Are Saying
Higher Education and Research Developer Resources
Self-Paced Online Course
Take a hands-on introductory course from the NVIDIA DLI to explore physics-informed machine learning with Modulus.
Access CourseTeaching Kit for Educators
A DLI Teaching Kit is available to qualified university educators interested in Physics-ML. Comprehensive and modular, the kit can help you integrate lecture materials, hands-on exercises, GPU cloud resources, and more into your curriculum.
Access Teaching KitOpen Hackathons and Bootcamps
Accelerate and optimize research applications with mentors by your side.
End-to-End AI for Science Hackathon GitHubUpcoming Open HackathonsIntroductory Resources
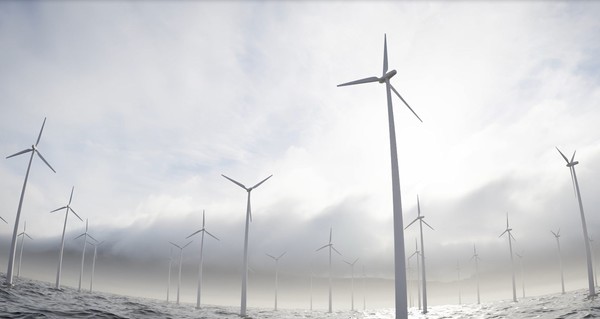
Using NVIDIA Modulus and Omniverse Wind Farm Digital Twin for Siemens Gamesa
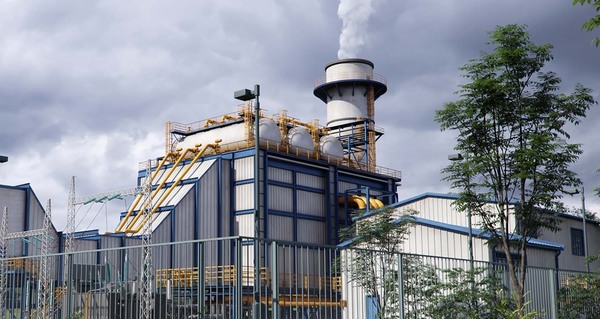
Siemens Energy Taps NVIDIA to Develop Industrial Digital twin of Power Plant in Omniverse and Modulus
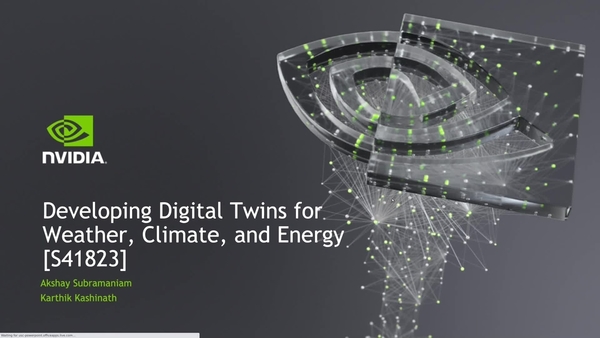
Developing Digital Twins for Weather, Climate, and Energy
Modulus Featured Content
Download the NVIDIA Modulus framework today.