What Is Conversational AI?
Conversational AI is the use of machine learning to develop speech-based apps that allow humans to interact naturally with devices, machines, and computers using audio. You use conversational AI when getting weather updates from your virtual assistant, when asking your navigation system for directions, or when communicating with a chatbot online. You speak in your normal voice and the device understands, finds the best answer, and replies with speech that sounds natural. However, the technology behind conversational AI is complex, involving multiple steps that require a massive amount of computing power and computations that occur in less than 300 milliseconds to deliver a great user experience.
How Does Conversational AI Work?
In the last few years, deep learning has improved the state of the art in conversational AI and offered superhuman accuracy on certain tasks. Deep learning has also reduced the need for deep knowledge of linguistics and rule-based techniques for building language services, which has led to widespread adoption across industries like telecommunications, unified communications as a service (UCaaS), retail, healthcare, and finance.
When you present an application with a question, the audio waveform is converted to text during the automatic speech recognition (ASR) stage. The question is then interpreted, and the device generates a smart response during the natural language processing (NLP) stage. Finally, the text is converted into speech signals to generate audio for the user during the text-to-speech (TTS) stage. Several deep learning models are connected into a pipeline to build a conversational AI application.
Over time, the size of models and number of parameters used in conversational AI models has grown. BERT (Bidirectional Encoder Representations from Transformers), a popular language model, has 340 million parameters. Training such models can take weeks of compute time and is usually performed using deep learning frameworks, such as PyTorch, TensorFlow, and MXNet. Models trained on public datasets rarely meet the quality and performance expectations of enterprise apps, as they lack context for the industry, domain, company, and products.
One approach to address these challenges is to use transfer learning. You can start from a model that was pretrained on a generic dataset and apply transfer learning to fine-tune it with proprietary data for specific use cases. Fine-tuning is far less compute intensive than training the model from scratch.
During inference, several models need to work together to generate a response—in only a few milliseconds—for a single query. GPUs are used to train deep learning models and perform inference, because they can deliver 10X higher performance than CPU-only platforms. This makes it practical to use the most advanced conversational AI models in production.
The Two Components of the Conversational AI Pipeline
1. Speech AI
a. Automatic Speech Recognition (ASR) or Speech-to-Text (STT)
b. Text-to-Speech (TTS) with voice synthesis
2. Natural Language Processing (NLP) or Natural Language Understanding (NLU)
Speech AI
Speech AI includes two key technologies: ASR and TTS. These are primarily used to provide a human-like voice interface to conversational AI applications such as virtual assistants.
Automatic Speech Recognition
ASR takes human voice as input and converts it into readable text. Deep learning has replaced traditional statistical methods, such as hidden Markov models and Gaussian mixture models, as it offers higher accuracy when identifying phonemes.
Popular deep learning models for ASR include Wav2letter, DeepSpeech, Listen, Attend, and Spell (LAS), Citrinet, Conformer, and more recently, Citrinet by NVIDIA Research. OpenSeq2Seq is a popular toolkit for developing speech applications using deep learning. Kaldi is a C++ toolkit that, in addition to deep learning modules, supports traditional methods like those mentioned above. GPU-accelerated Kaldi solutions can perform 3,500X faster than real-time audio and 10X faster than CPU-only options.
In a typical ASR application, the first step is to extract useful audio features from the input audio and ignore noise and other irrelevant information. Mel-frequency cepstral coefficient (MFCC) techniques capture audio spectral features in a spectrogram or mel spectrogram.
Spectrograms are passed to a deep learning-based acoustic model to predict the probability of characters at each time step. During training, the acoustic model is trained on datasets (e.g., LibriSpeech ASR Corpus, Wall Street Journal, TED-LIUM Corpus, Google Audio set) consisting of hundreds of hours of audio and transcriptions in the target language. The acoustic model output can contain repeated characters based on how a word is pronounced.
The decoder and language model convert these characters into a sequence of words based on context. These words can be further buffered into phrases and sentences, and punctuated appropriately before sending to the next stage.
There are also other training options, such as word-piece encoding and sentence-piece encoding, that we can use to train neural acoustic models to predict characters, words, and sentences. For language models, you can use either an n-gram language model or a neural rescoring language model to determine the correct output sentence.
To save time, pretrained ASR models, the NVIDIA TAO Toolkit, NVIDIA® Riva, training scripts, and performance results are available in the NVIDIA NGC™ catalog.
To learn more, refer to the resources below:
- Automatic Speech Recognition Starter Kit
- Building Transcription and Entity Recognition Apps Using NVIDIA Riva (blog)
- Build Domain Specific Speech Recognition Models on GPUs (blog)
- Develop Speech Recognition Models with NVIDIA's NeMo Framework (blog)
- Accelerating Automated Speech Recognition On-Demand Webinar (on-demand webinar)
- Automatic Speech Recognition Sessions and Demos
Text-To-Speech (TTS)
The last stage of the conversational AI pipeline involves taking the text response generated by the NLU stage and changing it to natural-sounding speech. This vocal clarity is achieved using deep neural networks that produce human-like intonation and a clear articulation of words. This step is accomplished with two networks. A synthesis network generates a spectrogram from text, and a vocoder network generates a waveform from the spectrogram. Popular deep learning models for TTS include RadTTS, FastPitch, HiFiGAN, Wavenet, Tacotron, Deep Voice 1, and Deep Voice 2.
Some of the open-source datasets for TTS are LJ Speech, Nancy, TWEB, and LibriTTS that have a text file associated with the audio. Preparing the input text for synthesis requires text analysis, such as converting text into words and sentences, identifying and expanding abbreviations, and recognizing and analyzing expressions. Expressions include dates, amounts of money, and airport codes.
The output from text analysis is passed into linguistic analysis for refining pronunciations, calculating the duration of words, deciphering the prosodic structure of utterance, and understanding grammatical information.
Output from linguistic analysis is then fed to a speech synthesis neural network model, such as FastPitch, which converts the text to mel spectrograms and then to a neural vocoder model like HiFiGAN to generate the natural-sounding speech.
NGC provides pretrained TTS models, along with training scripts and performance results. GPU-accelerated FastPitch and HiFiGAN can perform inference 12X faster on NVIDIA A100 Tensor Core GPUs than Tacotron2 and WavGlow on NVIDIA V100 Tensor Core GPUs.
To learn more, refer to the resources below:
Building Speech AI Applications
Get started with developing real-time speech AI pipelines for your conversational AI application.
Download E-book
Natural Language Processing (NLP) or
Natural Language Understanding (NLU)
NLU takes text as input, understands context and intent, and generates an intelligent response. Deep learning models are applied for NLU because of their ability to accurately generalize over a range of contexts and languages. Transformer-based models, such as BERT, revolutionized progress in NLU by offering accuracy comparable to human baselines on benchmarks like the Stanford Question Answering Dataset (SQUAD) for question answer (QA), entity recognition, intent recognition, sentiment analysis, and more.
In an NLU application, the input text is converted into an encoded vector using techniques such as Word2Vec, TF-IDF vectorization, and word embedding. These vectors are passed to a deep learning model, such as a recurrent neural network (RNN), long short-term memory (LSTM), and Transformer to understand context. These models provide an appropriate output for a specific language task like next-word prediction and text summarization, which are used to produce an output sequence.
However, text-encoding mechanisms, such as one-hot encoding and word-embedding can make it challenging to capture nuances. For instance, the bass fish and the bass player would have the same representation. When encoding a long passage, they can also lose the context gained at the beginning of the passage by the end. BERT is deeply bidirectional and can understand and retain context better than the other text encoding mechanisms. The key challenge with training language models is the lack of labeled data. BERT is trained on unsupervised tasks and generally uses unstructured datasets from books corpus, English Wikipedia, and more.
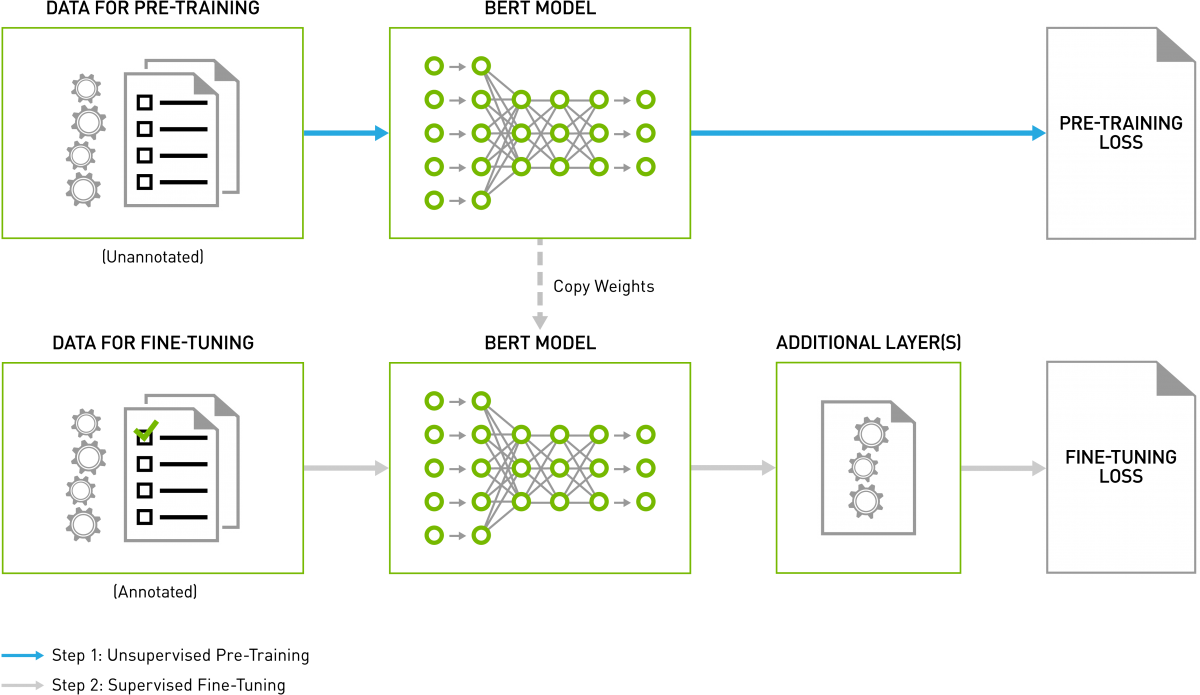
BERT-Large has 345 million parameters, requires a huge corpus, and can take several days of compute time to train from scratch. A common approach is to start from pretrained BERT, add a couple of layers to your task, and fine-tune on your dataset (as shown in figure 4). Available open-source datasets for fine-tuning BERT include SQuAD, Multi-Domain Sentiment Analysis, Stanford Sentiment Treebank, and WordNet.
GPU-accelerated BERT-base can perform inference 17X faster with NVIDIA T4 Tensor Core GPUs than CPU-only solutions. The ability to use unsupervised learning methods, transfer learning with pretrained models, and GPU acceleration has enabled widespread adoption of BERT in the industry.
NGC provides several pretrained NLP models including BERT, NVIDIA NeMo, NVIDIA TAO Toolkit and Riva, along with training scripts and performance results.
To learn more, refer to the resources below:
Industry Applications for Conversational AI
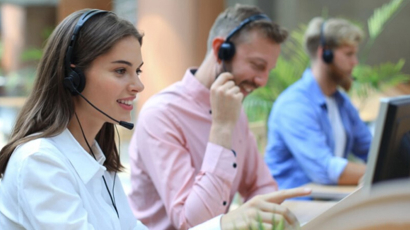
Telecommunications
Call centers are the telecom industry’s backbone, handling an average of 2 billion hours of phone calls daily. Enabling agents at these call centers will save both time and money. Businesses that integrate conversational AI can assist call center agents with real-time recommendations and insights. For instance, by using ASR, customer calls can be transcribed in real time, analyzed, and routed to the appropriate person to assist in resolving the query. Additionally, organizations can use these generated transcriptions to understand customer’s sentiment.
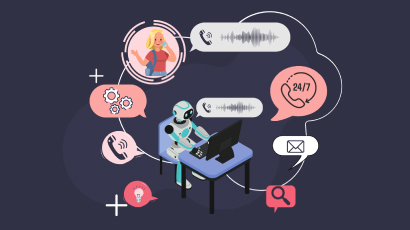
Financial Services
Conversational AI applications are enhancing customer service functions at financial institutions by helping users autonomously manage simple tasks, such as making payments ands managing refunds. It also aids in fraud detection by identifying anomalies from past experiences, activities, and behaviors. In the insurance sector, AI assistants accelerate claims by engaging customers with dynamic conversations.
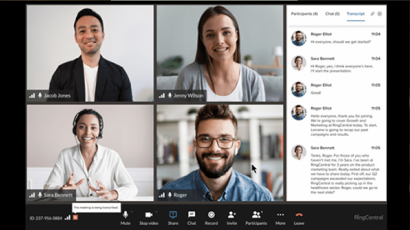
UCaaS
Unified communications as a service (UCaaS) offers a wide range of applications and services in the cloud for communication and collaboration. One of the key areas in which UCaaS solutions are used is audio and video conferencing. Speech recognition and neural machine translation can be used in video conferencing apps to generate meeting notes and translation in real time, allowing for smoother conversations with regional speakers. Companies can also incorporate virtual assistants into their web conferencing applications to help with scheduling and facilitating meetings.
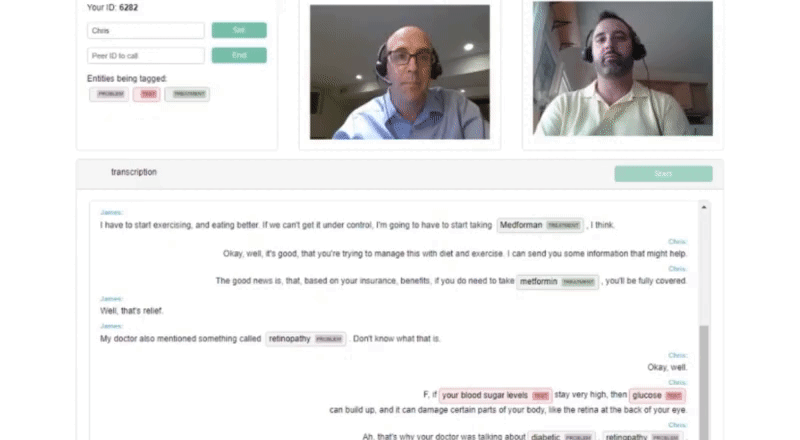
Healthcare
Conversational AI is making healthcare more accessible and improving the patient experience. ASR models are being used for transcribing physician notes, capturing physician and patient consultations, and converting speech to text for clinical documentation. NLU is being utilized for chatbots that assist patients with selecting the right health insurance plan, onboarding, and appointment scheduling. NLU is also used to extract relevant medical information from a large volume of unstructured data to help with medical diagnoses. And TTS models help people with reduced vision or learning disabilities by reading medical information aloud from websites, medication leaflets, and other digital content.
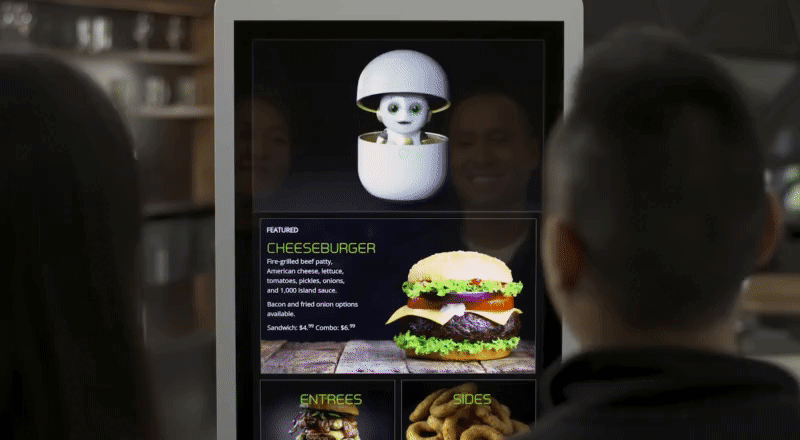
Retail
Chatbots are commonly used in retail applications to accurately understand customer queries and generate responses and recommendations. AI virtual assistants allow customers to shop online using only their voice, bridging the gap between physical and virtual shopping and improving efficiencies in store operations. NLP is also used for mining customer feedback and sentiment analysis, leading to higher customer retention rates.