NVIDIA Merlin
NVIDIA Merlin™ is an open-source framework for building high-performing recommender systems at scale.
Designed for Recommender Workflows
NVIDIA Merlin empowers data scientists, machine learning engineers, and researchers to build high-performing recommenders at scale. Merlin includes libraries, methods, and tools that streamline the building of recommenders by addressing common preprocessing, feature engineering, training, inference, and deploying to production challenges. Merlin components and capabilities are optimized to support the retrieval, filtering, scoring, and ordering of hundreds of terabytes of data, all accessible through easy-to-use APIs. With Merlin, better predictions, increased click-through rates, and faster deployment to production are within reach.
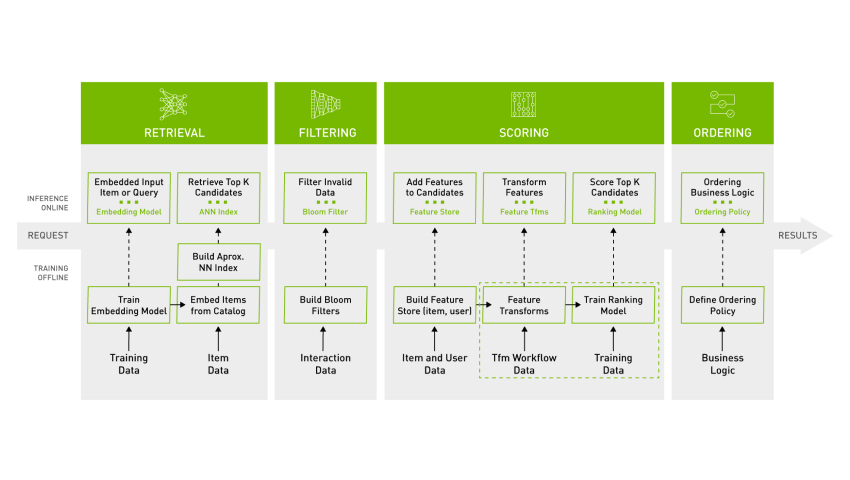
Interoperable Solution
NVIDIA Merlin, as part of NVIDIA AI, advances our commitment to support innovative practitioners doing their best work. NVIDIA Merlin components are designed to be interoperable within existing recommender workflows that utilize data science, machine learning (ML), and deep learning (DL) on CPUs or GPUs. Data scientists, ML engineers, and researchers are able to use single or multiple components, or libraries, to accelerate the entire recommender pipeline—from ingesting, training, inference, to deploying to production. NVIDIA Merlin's open-source components simplify building and deploying a production-quality pipeline.
Merlin Models
Merlin Models is a library that provides standard models for recommender systems and high quality implementations from ML to more advanced DL models on CPUs and GPUs. Train models for retrieval and ranking within 10 lines of code.
Try it today:
Merlin NVTabular
Merlin NVTabular is a feature engineering and preprocessing library designed to effectively manipulate terabytes of recommender system datasets and significantly reduce data preparation time.
Merlin HugeCTR
Merlin HugeCTR is a deep neural network framework designed for recommender systems on GPUs. It provides distributed model-parallel training and inference with hierarchical memory for maximum performance and scalability.
Merlin Transformers4Rec
Merlin Transformers4Rec is a library that streamlines the building of pipelines for session-based recommendations. The library makes it easier to explore and apply popular transformers architectures when building recommenders.
Merlin Distributed Training
Merlin provides support for distributed training across multiple GPUs. Components include Merlin SOK (SparseOperationsKit) and Merlin Distributed Embeddings (DE). TensorFlow (TF) users are empowered to use SOK (TF 1.x) and DE (TF 2.x) to leverage model parallelism to scale training.
Try it today:
Merlin Systems
Merlin Systems is a library that eases new model and workflow deployment to production. It enables ML engineers and operations to deploy an end-to-end recommender pipeline with 50 lines of code.
Try it today:
Built on NVIDIA AI
NVIDIA AI empowers millions of hands-on practitioners and thousands of companies to use the NVIDIA AI Platform to accelerate their workloads. NVIDIA Merlin, is part of the NVIDIA AI Platform. NVIDIA Merlin was built upon and leverages additional NVIDIA AI software within the platform.
RAPIDS
RAPIDS is an open-source suite of GPU-accelerated python libraries, enhancing performance and speed across data science and analytics pipelines entirely on GPUs.
Try it today:
cuDF
cuDF i is a Python GPU DataFrame library for loading, joining, aggregating, filtering, and manipulating data.
Try it today:
NVIDIA Triton Inference Server
Take advantage of NVIDIA Triton™ Inference Server to run inference efficiently on GPUs by maximizing throughput with the right combination of latency and GPU utilization.
Try it today:
Production Readiness with NVIDIA AI Enterprise
NVIDIA AI Enterprise accelerates enterprises to the leading edge of AI with enterprise-grade security, stability, manageability, and support while mitigating the potential risks of open source software.
- Secure, end-to-end AI software platform
- API compatibility for AI application lifecycle management
- Management software for workloads and infrastructure
- Broad industry ecosystem certifications
- Technical support and access to NVIDIA AI experts
Learn More
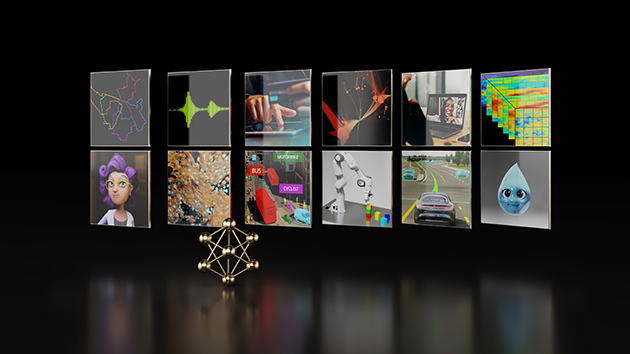
Resources
Recommender Systems, Not Just Recommender Models
Learn about the 4 stages of recommender systems pattern that covers the majority of modern recommenders today.
GTC Spring 2022 Keynote: NVIDIA Merlin
Watch as NVIDIA CEO Jensen Huang discusses how recommenders personalize the internet and highlights NVIDIA Merlin use cases from Snap and Tencent's WeChat.
Optimizing ML Platform with NVIDIA Merlin
Discover how Meituan optimizes their ML Platform using NVIDIA Merlin.
Next Gen Recommenders with Grace Hopper
Learn more about Grace Hopper Superchip and how it provides more memory and greater efficiency for recommender workloads.
Explore Merlin resources
Take this survey to share some information about your recommender pipeline and influence the NVIDIA Merlin roadmap.